Faculty of Information and Communication Technology (Kampar Campus), UTAR xiii Diagram 2.8.3 Table of 3 grouped with their respective mean and standard. Faculty of Information and Communication Technology (Kampar Campus), UTAR xiv Diagram 4.3.1 Shows the used examples of synthetic datasets 42. 44 Diagram 4.4.3 Shows the function defined in the program 45 Diagram 4.4.4 Shows the main function of the program 46 Diagram 4.4.5 Shows sample output of classification and domain.
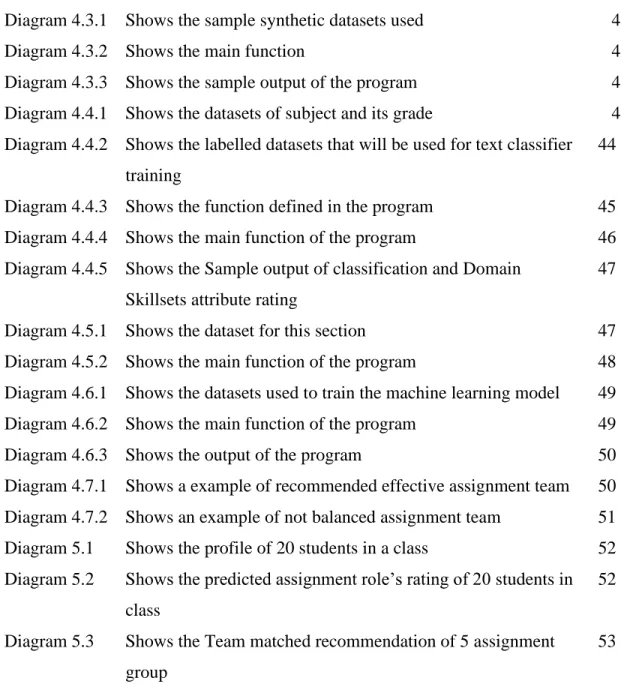
Introduction
- Problem Statement and Motivation
- Background
- Project Objectives
- Proposed work
- Background Information
- Impact, significance and contribution
This paper will discuss about a widespread method of student profiling for team match recommendation, which is to use the generated student profile to help recommend team matching. This is the core idea of this research paper, where in this paper few characteristics attributes will be determined, then a profiling of the students will be done. The data collection method will also be determined to collect different data from different data sources to determine the evaluation of these attributes of the defined characteristics.
Faculty of Information and Communication Technology (Kampar Campus), UTAR 3 who complete different scope of work, we can then use it as a reference to help in the implementation of team matching recommendation, where different individuals who are good in different aspect of work will be grouped together to a more balanced assignment team. Then different data collection methods will be used for collecting data respectively for each feature. Machine learning algorithms will be used to learn and train the data sets to create a model for prediction.
The most crucial phase of all this will be the data collection phase where all data will be collected from different data sources to determine the value of each characteristic in the profiling process. The labeled data will be used to fit the machine learning algorithms for training and finally come up with a model to perform prediction.
Literature Review
- Predict student academic performance based on self-regulating characteristics
- Learning Human Behavioral Profiles in a Cyber Environment
- Use of Personality Profile in Predicting Academic Emotion based on Brainwaves Signals
- Academic Advising System Using Data Mining Method for Decision Making Support
- Teacher Assessment and Profiling using Fuzzy Rule based System and Apriori Algorithm
- Clustering and Profiling Students According to their interactions with an Intelligent
- Data-driven learner profiling based on clustering student behaviors: Learning
Faculty of Information and Communication Technology (Kampar Campus), UTAR 8 The answered questionnaires of each student will then be calculated by calculating the average values of each dimension. Faculty of Information and Communication Technology (Kampar Campus), UTAR 9 According to Julieta Noguez, Luis Neri, et.al (2016), the validation shows very good agreement between the profiles assigned by system and those observed by students. The algorithm of data mining used in this study is K-means clustering algorithm which is used to group students according to their similar characteristic.
Faculty of Information and Communication Technology (Kampar Campus), UTAR 13 Diagram 2.4.2 shows the output of K average algorithms (Source of: Riah F. From the result of grouping, the model for decision support is formed by analyzing statistically based on the result grouping Faculty of Information - and Communication Technology (Kampar Campus), UTAR 14 The diagram above is the flow diagram of the proposed system model where the user will be the student and they can go to the assessment system to fill in the questionnaire about the respective teacher they have chosen.
Student feedback will eventually feed into the fuzzy rule base system and profile the system based on the student questionnaire. Faculty of Information and Communication Technology (Kampar Campus), UTAR 15 From this research we can know that the apropri method is a good way to find correlation between different data and the relationships between them. Then MLFS is used to be informed about the importance of each feature by calculating the total ranking score of all defined features.
Faculty of Information and Communication Technology (Kampar Campus), UTAR 16 Diagram 2.6.2 shows the summary of ranking results of all features (Source from: Wei-Xiang Liu and Ching-Hsue Cheng (2016)). Based on the table above, the accuracy looks better and better when more and more low impact features are removed. Then the Bi-Modal LSTM model will be used to encode visual and audio modes with temporal modeling.
Faculty of Information and Communication Technology (Kampar Campus), UTAR 18 Diagram 2.7.1 showing the Deep Bi-modal LSTM model architecture (Source of: Karen Yang and Nao Glaser (2017)). Faculty of Information and Communication Technology (Kampar Campus), UTAR 20 profile is created which helps them to distinguish different types of learners based on the 12 variables. Faculty of Information and Communication Technology (Kampar Campus), UTAR 21 They then use mean shift clustering to identify 5 possible clusters in the data and set up K-means clustering to find 5 different groups of students.

System Design
- Overview
- Profiling
- Assignment team roles
- Implementation Issues and challenge
These attributes will be calculated in terms of a numerical value and the data sets collected from the concerned individuals will determine the scores of these attributes. In this research, UTAR will be the experimental training ground for the research, and all these data sources are also from UTAR. For the domain skillset attribute, the data collected will be the student's academic record, which records the grade for each course or course the student has taken, the course grade determines the skill the student has.
Students' grade in both these subjects will be the determination of the value of learnability attribute. However, for leadership characteristics, we will only focus on the leadership component of the USSDC report. Faculty of Information and Communication Technology (Kampar Campus), UTAR 24 Productivity, the data will be collected from student-completed assignment.
Three important criteria will be used as the data sets needed to calculate student productivity, which are assignment grade, assignment due date, and assignment. Each attribute of a student profile will range from 1 to 5 representing the rating of player characteristics in each attribute. The calculation part will involve the calculation of the grade value difference between the related subject according to the list of subject relationship rules above.
Then, based on all the sites collected, a calculation will be performed to determine the rating of exploratory attribute value. Productivity attribute assessment will be determined through the student's assignment start date, submission date and assignment grade to calculate the rate for. To obtain the domain skill set of a student, the method proposed in this research is to determine it by getting the grade for the subject.
In this paper, a subject from the computer science degree at UTAR will be used as an example. The average grade of all subjects in these 7 areas will be calculated, which will come out with the average value of the grade of each area. The final Domain Skillset attribute score will be the average of each domain's average score.
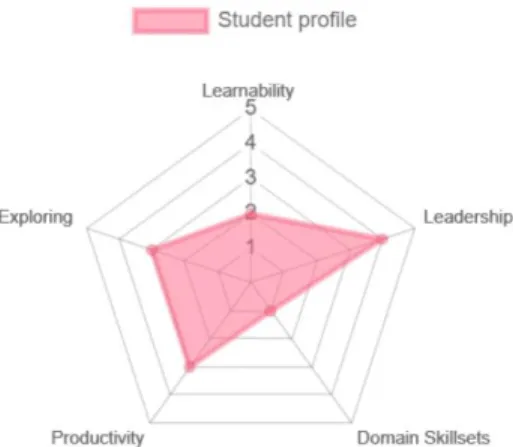
Methodology
- Exploring Attribute
- Learnability Attribute
- Productivity Attribute
- Domain Skillsets
- Leadership Attribute
- Machine learning
- Team matching recommendation
Faculty of Information and Communication Technology (Kampar Campus), UTAR 36 The website which category falls under "Education" and "Technology" will both. Faculty of Information and Communication Technology (Kampar Campus), UTAR 37 Using the formula mentioned in chapter 3 system design. The above diagram shows the datasets for the subject found in UTAR Computer Science program structure and its grade.
Faculty of Information and Communication Technology (Kampar Campus), UTAR 45 Swticher(): This function is defined to perform switching case functionality where it consists of cases of different grade for A+ to C and their respective grade value. Faculty of Information and Communication Technology (Kampar Campus), UTAR 49 Diagram 4.6.2 shows the main function of the program. Above diagram shows the output of the prediction which is the performance rating of each roles.
Faculty of Information and Communication Technology (Kampar Campus), UTAR 50 As you can see from the diagram above, the recommended assignment team consists of 4 students who each had a higher rating of at least one of a role that is much more balanced and effective team.
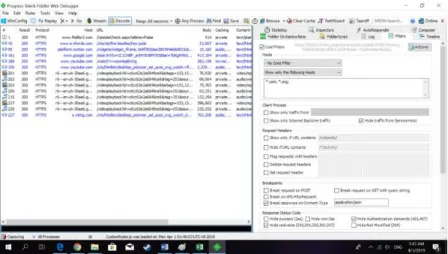
Implementation and Testing
Conclusion
Faculty of Information and Communication Technology (Kampus Kampus), UTAR 54 all experimental, all methods implemented are just an idea, maybe inaccurate. The idea proposed in this research may be a possible solution to help solve the problem of group formation for university assignments. With the help of this research method, it is possible to recommend the formation of a much more effective and balanced team.
Currently, the method proposed to perform team matching recommendation is artificially matched by referring to the student's predicted assignment role abilities. This entire process can all be automated without involving a human, using a deep learning technique to do so. With the help of deep learning, the entire team matching process can be automated and thereby predicted.
RNN (Recurrent Neural Networks) may be the algorithm of choice for use in team matching because RNN can meaningfully capture relationships across inputs. Use of Personality Profile in Predicting Academic Emotion based on Brainwaves Signals and Mouse Behavior, 2011 Third International Conference on Knowledge and Systems Engineering, pp. Faculty of Information and Communication Technology (Kampar Campus), UTAR 56 8) Matthew Mayo, 2018. Frameworks for Approaching the machine learning process.
Grouping and profiling of students according to their interactions with an intelligent teaching system that promotes self-regulated learning. Place a checkmark in the left column after reviewing your report in relation to the corresponding item. All references in bibliography are cited in the thesis, especially in the literature review chapter.