The author has also granted permission to the University to retain or make a digital copy for similar use and for the purpose of digitally preserving the work. This allowed the author to discover that machine learning is very prevalent in UAE supply chains with artificial intelligence specifically lacking.
Research background
Each of these can lead to supply chain complexity in environments that are already uncertain. AI enables the implementation of predictive approaches that aid rapid evaluations and effectively minimize disruptive and risky events that may occur across the supply chain.
UAE logistics industry
Research on the matter shows that the nation's logistics sector is likely to contribute eight percent to the nation's economy, a five percent increase from its previous rate (Cioffi, et al., 2020). In fact, this sector has regularly outperformed a number of other important industries and is currently considered one of the most significant enablers of the nation's economic diversification efforts (Cioffi, et al., 2020).
Research questions
Despite the economic headwind swirling across the Gulf and the economic conditions of recent years, the logistics industry remains one of rapid development and progress.
Research aim
Research objectives
Examine the supply chain-based trends of automation in relation to machine learning and artificial intelligence.
Research process
Dissertation structure
Introduction
Artificial intelligence and design
Application of AI in value creation
It has been due to artificial intelligence that the automation process has been able to advance by leaps and bounds in the last few years. One of the advanced branches of artificial intelligence includes robotics – something that plays an important role in manufacturing (Shah, et al., 2020). A good example of the way artificial intelligence helps in manufacturing would be the manufacturing process of semiconductor chips that is enabled by artificial intelligence.
Companies in the utility sector use artificial intelligence to maintain their facilities and networks. Artificial intelligence forecasts are so reliable that Otto, when expanding his inventory, can anticipate orders without relying on human intervention (Hofmann, et al., 2019).
Machine learning
- Supervised learning
- Perceptron
- Adaptive linear neuron (AdaLiNe)
- K-nearest neighbors (KNN)
- Unsupervised learning
- Density-based spatial clustering of applications with noise (DBSCAN)
- Reinforcement learning
- Demand forecasting with machine learning
The schedules of the forwarded calendars were not established, which meant that the algorithm was not used at work. Based on the weighting coefficients of the algorithm, the function gives a value, the so-called price, which is negative for the operation of the algorithm. In learning, the optimization of weight coefficients is based on the probability that the algorithm's class prediction is correct (Gray-Hawkins & Lăzăroiu, 2020).
Since the algorithm calculates the probability, the threshold value of the quantizer can be set based on the proportion of free time present in the training data. Based on the similarity of the objects, the algorithm divides the objects into groups to find patterns in the dataset.
Key issues identified from the reviewed literature
As the author has analyzed the available technologies of artificial intelligence and machine learning, there seems to be a lack of knowledge about what is the minimum level of technology required for complete automation of supply chain methods and therefore this paper will evaluate this aspect.
Chapter Summary
Introduction
Thus, due to the nature of this study and with the aim of achieving the set goal, we decided to use a quantitative methodology as a methodology. Although a mixed methods approach would have been better, the author lacked the time and resources necessary to use such an approach, and it was recommended that further studies use such approaches. It should also be understood that a quantitative approach is much more useful than a qualitative approach in this regard, as this approach allows for the collection of a large enough sample population for analysis purposes that offers generalizable results and findings.
This allowed the author to offer insight into the matter as well as explore it in detail. Conversely, a qualitative focus would have involved the use of a smaller sample population and more exploration of the human impact of the technologies at hand, thus reducing the generalizability of the findings and their statistical significance.
Justification of the method
In turn, according to Ørngreen & Levinsen (2017), Zangirolami-Raimundo, Echeimberg & Leone (2018) and Nayak & Singh (2021), the quantitative methodology is sound and offers advantages, as it has been a successful approach in other studies . So it is not that the quantitative methodology is better than the others, but rather that it is more suitable for the current research. In addition, the use of different methods can allow a better understanding of the phenomena and thus obtain deeper and more secure results from the research in question.
In other words, considering the purpose of the study, and the results intended to be achieved, this methodology enabled the author to guarantee the validity and reliability of the data, even while others may have been helpful, would they the goals and objectives of the current work. In this line of thought, there are authors, for example, Nayak & Singh (2021) who highlight the problems of using this method and claim that the use of different research methods is also based on different assumptions, among other things, about social reality. and nature of data collection.
Data Collection Tool
Given that reality is complex, we have to use different methods to analyze this same reality. Ørngreen & Levinsen (2017) warn that despite the advantages of combining methods, this procedure presents some disadvantages and even a great challenge for the researcher when he wants to master the technique well.
Sample
Survey
Data analysis
Validation and verification
Chapter summary
In particular, it can be seen here that the majority, almost fifty percent, of the participants were between the ages of twenty-eight and thirty-seven years. Although many of the respondents said they were very literate when it came to technology, a surprising number of participants, totaling fifteen percent, said they were somewhat literate when it came to technology. Specifically, seventy-two percent of respondents said they were very technologically literate, while thirteen percent said they were competently literate and fifteen percent said they were somewhat technologically literate.
In this regard, the majority of participants were male, with seventy-six percent of them being male, while the rest were female. Here it can be seen that the majority had three to six years of work experience, while twenty-four percent of the participants had six to twelve years of work experience.
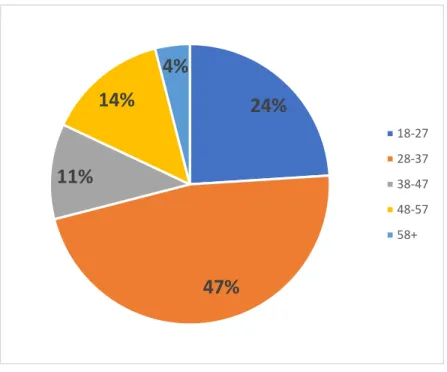
Trends in supply chain
After this, the participants were asked about the integration of machine learning in their company. Here, Figure 3 shows that more than 70 percent of respondents' companies had fully integrated machine learning, receiving similar opposing responses when participants were confronted with the idea that their companies were not integrating machine learning at all. This further illustrates that machine learning and similar automations were actively implemented in the supply chain.
Finally, more than ten percent indicated that their organization did not integrate machine learning at all. Overall, forty-three percent of participants collectively agreed that the supply chain process had become dependent on automation to perform its tasks and processes, while thirty-nine percent disagreed with this perspective.

Application of artificial intelligence and machine learning
The responses herein show that the participants' organizations focused on automating tasks, but also ensured that there was a person responsible for overseeing the automation to ensure no errors occurred. Finally, participants were asked whether or not their companies had primarily used artificial intelligence and machine learning technologies to automate tasks such as inventory management - as previous answers also pointed to the use of these technologies in this regard and the literature on the matter shows also see that this is quite common in supply chains. By responding to this, the respondents collectively agreed, demonstrating that this was the case, consistent with the literature on this issue.
This illustrates that automation was mainly used to ensure that simple tasks that did not require supervision could be automated. In this sense, automation was primarily used to complement human workers and ensure that they could improve their performance and operations, thereby improving the overall supply chain through the integration of such automated technologies.
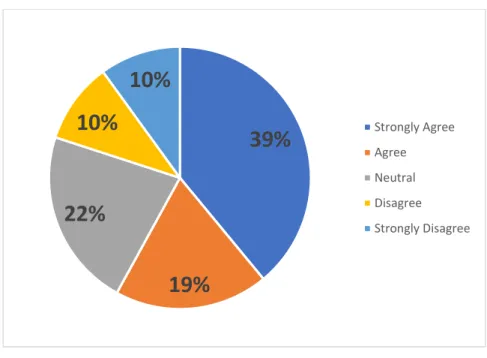
Supply chain efficiencies
Notably, nearly eighty percent of participants had stated this and indicated that their supply chains were significantly improved as a result of such automation technologies. The reverse of this question item was then asked at the end of the survey to assess whether or not the participants' supply chain, in their opinion, had become inefficient through the implementation of automation technologies. However, this was not the case as those who had agreed with the penultimate question collectively disagreed with the last question they were asked.
Introduction
The main type used by respondents was one that did not require human supervision and still had human workers monitoring their performance and results. This allowed these companies to ensure that these technologies worked together with their workers and that they did not make any mistakes in their operation or performance.
Recommendations
Thus, it is important for those implementing such technologies to ensure that they take into account such factors as well as evaluate the overall costs associated with implementing either machine learning or artificial intelligence.
Suggestions for Future Research
Supply chain risk management and artificial intelligence: state of the art and future research directions. Applications of artificial intelligence in supply chain management and logistics: Focus on recognition for supply chain execution. Exploring the role of artificial intelligence in managing agricultural supply chain risk to address the effects of the COVID-19 pandemic.
Apply machine learning to the dynamic selection of supply policies in rapidly changing supply chain environments. How can e-grocers use artificial intelligence based on technology innovation to improve supply chain management?