To solve the problem and to show or report real data, this work was started and created an impactful result that will help others to take the right steps to reduce gaming addiction. Spend most or all of your free time playing video games, lose productivity at work or school, choose to play video games over sleep, socializing, or other activities, lie or keep your video game use a secret, and avoid or stop participating to other activities to spend more time playing video games are early indicators or warning signs of video game addiction. While some people may experience the signs of video game addiction quickly, for the majority the process is more subtle.
Finally, the use of data science to predict and prevent gambling addiction is likely to increase in the future. In addition, there may be a greater need for research into the prediction and prevention of gaming addiction due to the popularity and availability of video games. New methods and strategies could be created as this field of study evolves to better predict and prevent gambling addiction.
Motivation
Objectives
Methodology
Rationale of the Study
Research Questions
Expected Output
Project Management and Finance
Research Layout
BACKGROUND STUDY
- Terminologies
- Related Works
- Comparative Analysis and Summery
- Scope of the Problem
- Challenges
Occasionally, playing digital games on a computer screen or mobile device can be considered just one of the various leisure activities available. Due to the accessibility and portability of modern media, many teenagers are observed spending several hours playing computer or internet games on their desktop, laptop, tablet or mobile device.[3] The popularity of video games has skyrocketed recently and it is now a billion dollar industry. Gaming disorder (GD) was recognized in the eleventh edition of the International Classification of Diseases after DSM-5 identified "online gaming disorder" as a possible illness that merited further investigation (American Psychiatric Association Although INTERNET ADDICTION is not currently a DSM IV diagnosis , DSM IV has been used to define it.need for fixation and dependence.
Young, who first coined the term Internet Addiction Disorder and listed the diagnostic criteria, is often used as a starting point for studies. In their analyzes of Internet addiction, researchers like Young replace the word "drug" with "Internet," reaching the same conclusion that Internet addiction also exhibits similar symptoms including tolerance, withdrawal, craving, and negative outcomes. of life. Based on comparisons with gambling addiction and compulsive shopping, both of which lack a physiological addiction, Walker's research would classify Internet addiction as an obsessive and compulsive activity.
However, little is understood about Internet addiction in general.[5] This essay introduces the computer game Co-Op World as a tool for child psychotherapy. The fact that our game gives the therapist a priori control over the behavior of the virtual player, in contrast to traditional computer games or traditional therapy, allows for a variety of targeted interactions related to reciprocity between the patient and the virtual player, which is an essential aspect of new system. If it is possible by chance, there will surely be many errors.
Machine learning method or classification algorithm can produce desired results and can be done by early computation. These are: unlabeled data usage for unsupervised learning and labeled data usage for supervised learning. The various supervised learning and unsupervised learning machine learning algorithms are listed in the table below.
This section describes the data preprocessing, attribute selection, algorithm classification, related work and challenges in this study.
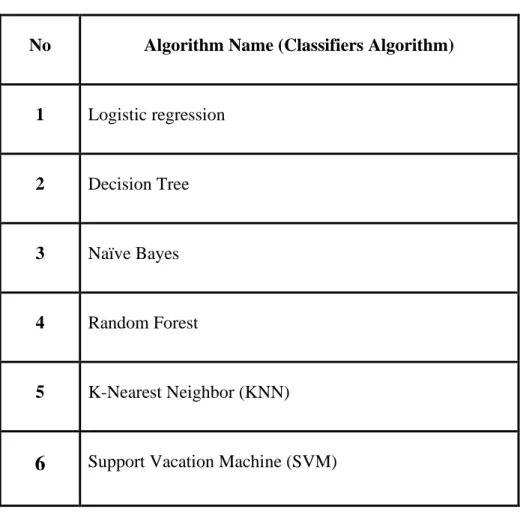
RESEARCH METHODOLOGY
- Research Subject and Instrumentation
- Data Collection Procedure
- Selective Algorithms
- Proposed Methodology
- Data Preprocessing
- Model Selection for classification problems
- Model Evaluation
- K-Fold Cross Validation
- Classification Report
- Implementation Requirements
Finally, with the help of the psychologists and online support, the combination of their various decisions in final leveling outputs with an emphasis on the opinion of the majority. DT is a tree where each internal node of the tree represents the attribute values, and the leaf node represents the decision. Random forest is a scalable, easy-to-use machine learning algorithm that produces a fantastic result most of the time, even without hyperparameter tuning.
Because of its simplicity and diversity, it is now one of the most widely used algorithms. It has an efficient method of calculating incomplete data that maintains precision in the absence of a significant part of the data. It calculates proximities between pairs of cases that can be used to cluster, find outliers or provide interesting views of the data (by scaling).
The above functionality can be applied to untagged files, resulting in unsupervised clustering, data views, and anomaly detection. This bag of data is used to achieve an unbiased ongoing calculation of classification error when adding trees to an area. The approximations are normalized at the end of the run by dividing by the number of trees.
The K-nearest neighbor criterion essentially reduces to forming a higher proportion of votes among the K most comparable occurrences with respect to a given one. Since KNN is a non-parametric computation, I will not retrieve the parameters for the model, but still recover a vector containing the elements of the test set characterizations. The majority of the scholar's field unit suffers from minimal or minimal levels of depression in their lifetime.
One of the most popular Supervised Learning algorithms used for classification and regression problems is the Support Vector Machine or SVM. Such extreme cases are square measure stated as support vectors and the rule is therefore noted because of the Support Vector Machine. One class is on one side and another class is on the other side of the room.
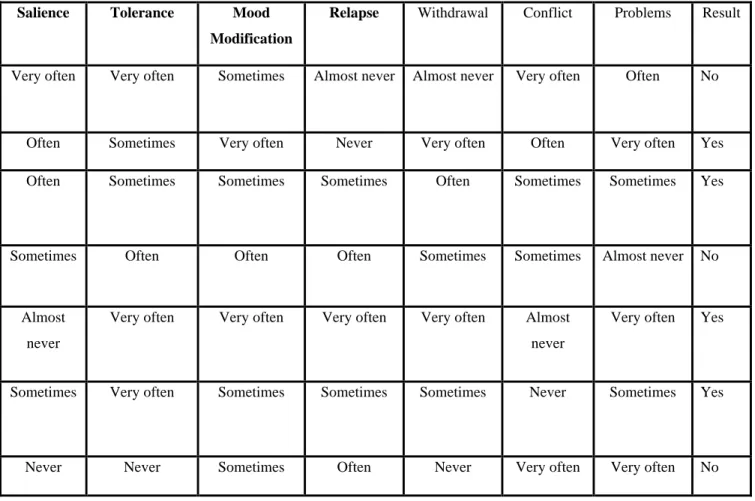
EX PERIMENTAL RESULTS AND DISCUSSION
Experimental Setup
Experimental Results and Analysis
Based on precision, the support vector machine algorithm has the highest precision, but its F1 score and recall and precision are also higher than any other selected algorithm. Thus, I can say that the testing algorithms using Random Forest show the highest accuracy during the testing process or period. This is a benchmark table that is mainly used to represent the performance of a machine learning model based on a set of test output data [15].
It checks the performance by calculating four terms such as True Positive (TP), True Negative (TN), False Positive (FP) and False Negative (FN). An N x N matrix, called the confusion matrix, where N is the total number of target classes, is used to evaluate the performance of the classification model. In the matrix, the actual target values contrast with those predicted by the machine learning model.
It is usually preferable to use the confusion matrix as an evaluation criterion for your machine learning model when working with an imbalanced data set.
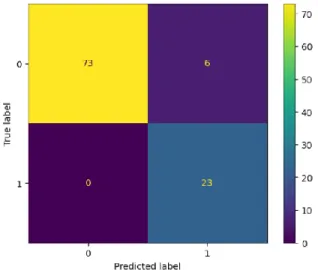
Discussion
IMPACT ON SOCIETY, ENVIRONMENT AND SUSTAINABILITY
Impact on Society
Impact on Environment
Ethical Aspects
Sustainability Plan
SUMMARY, CONCLUSION, RECOMMENDATION, AND IMPLICATION FOR FUTURE RESEARCH
- Summary of the Study
- Conclusion
- Recommendation
- Implication for Further Research
Use of big data and machine learning techniques: With more and more data available on the behavior and demographics of players in the game, big data and machine learning techniques can be used to analyze addiction and make predictions. Development of intervention and prevention strategies: Based on the results of the study, recommendations can be made for intervention and prevention strategies for individuals at risk of addiction, such as providing support and resources to help them manage their play time and reduce risk of addiction. Collaboration with game developers: To have a broader impact, research should include collaboration with game developers to design games that are less likely to lead to addiction.
Finally, it is important to note that gaming addiction is a complex and multifactorial problem that requires a multidisciplinary approach, so it is important to keep in mind that these recommendations should not be considered as the only solution. In the previous parts, finding the addictive level of the games was not much difficult, but now the strategy has changed, so the addictive level has also changed. From this part, you can understand the new strategies of the game developers and the level of addiction.
The previous works showed me a way to start the research work and the test criteria. I added the new methods using psychological knowledge and new testing algorithms to complete my research. They can also easily find out the dependency level by changing or adding new algorithms in their work.
Unsal Barlas, "Investigating the Level of Online Gaming Addiction, Sociodemographic Characteristics, and Social Anxiety as Risk Factors for Online Gaming Addiction in Middle School Students," Community Mental Health Journal, vol. Pasa, "Parenting and child's (five years to eighteen years) digital game addiction: A qualitative study in the northwestern part of Bangladesh," Computers in Human Behavior Reports, vol. Vimukthi, "Impact of Mobile Game Addiction on Sri Lankan Teenagers: An Exploratory Study," Asian Journal of Education and Social Studies, pp.
Chung, “Socioecological factors influencing adherence to fluid restriction among Korean patients receiving hemodialysis: a qualitative study,” Journal of Transcultural Nursing , vol. Chan, “Understanding massively multiplayer online role-playing addiction: a hedonic management perspective,” Information Systems Journal, Jun. Khaledi-Paveh, "Psychological problems as the main cause of fatigue in clients undergoing hemodialysis: A qualitative study," International Journal. of Nursing Sciences, vol.
Pamuk, “Opinions of hemodialysis and peritoneum patients regarding depression and psychological problems they experience: A. Lin, “Gaming addiction is involved in mental illness and medical care is a challenge: Textual and discourse analysis,” Fortune Journal of Health Sciences, vol .Gu, "Attraction and Addictive Factors of Internet Games for Older Adults: A Qualitative Study," Lecture Notes in Computer Science, p.
Wölfling et al., “Efficacy of short-term treatment of Internet and computer gaming addiction,” JAMA Psychiatry, vol. Yang, “Chinese Students' Behavior and Experiences of Internet Gaming Addiction: A Qualitative Case Study of CCNU via NVivo,” IEEE Xplore, Dec. TYSON, “The Concept of Transference in Child Psychoanalysis,” Journal of the American Academy of Child Psychiatry , vol.
Salkin, “Engagement with electronic screen media among students with autism spectrum disorders,” Journal of Autism and Developmental Disorders, vol.