PROJECT MANAGEMENT & EDITORIAL OFFICE Ben Gesing, Gianmarco Steinhauer, Michel Heck DHL Customer Solutions & Innovation. Just as the relational database found its way into mainstream business operations around the world – providing better ways to store, retrieve and organize information – AI is now following a similar path.
Origin & Definition of AI
Two terms in particular are important to understanding AI – machine learning, which is a subset of AI, and deep learning, which is a subset of machine learning, as depicted in Figure 3. In simplified form, Figure 5 shows how deep learning algorithms can distinguish between the content of an image, as well as where the elements of the image are in relation to each other, by analyzing pixel data alone.

How Machines Learn: Three Components of AI
Once an AI system has collected data through detection, it processes that information by applying a learning framework to generate insights from the data. Reinforcement learning takes place when the AI system not only has the task of processing the available input, but also of learning the 'rules of the game'.
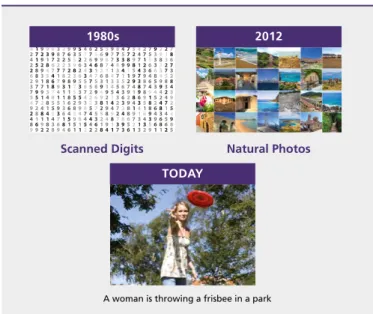
Trends Accelerating AI
This cycle of dependence on and appreciation of technology is central to the future trajectory of AI. While the current development of AI-based systems is primarily centered in the United States and secondarily China, the growth of AI is a truly global phenomenon.

Challenges & Risks
Today, about 60% of corporations in the Forbes Global 2000 are either carefully evaluating AI or using it productively by tailoring solutions to fit their specific business context and improving and extending proven solutions. As seen in Figure 18, in a McKinsey survey of 1,300 CEOs in 2016, only 20% of enterprises are already productively using AI technologies at scale or in their core business. Industry sectors leading AI adoption are those with core products or services centered on the technology.
One such example is the growth of a mid-skilled workforce to operate and manage the fleet of flexible, collaborative non-industrial robots shown in Figure 20. Another indirect effect of increased automation will be an increase in the value of human touch and connection.
Why Logistics? Why Now?
Logistics companies are uniquely positioned to benefit from applying artificial intelligence in almost every aspect of the supply chain. One of the most underutilized assets in the industry is the vast amount of data that supply chains generate on a daily basis. Additionally, as many logistics companies around the world embrace digital transformation, moving away from legacy enterprise resource planning systems to advanced analytics, increased automation, and hardware and software robotics and mobile computing, as seen in Figure 21, the next obvious step in the increasingly digital supply chain is to use AI.
There is another indicator that now is the right time for artificial intelligence to flourish - and that is the state of its acceptance in the world today. Eventually, when the value of artificial intelligence is proven in a commercial context, it will come to the industrial environment.
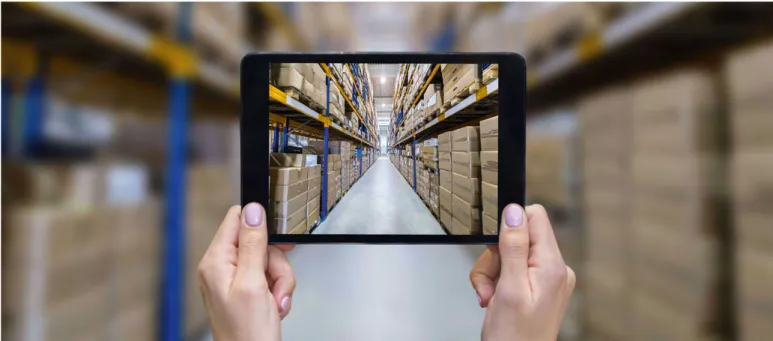
Consumer AI: Ambient Assistance in Everday Life
AI offers the opportunity to develop highly personalized products and services for customers on a large scale. Today, 3.2 billion of the 4 billion internet users or 79% of the global internet population are active on social media.20 The unstructured content on social platforms, blogs and other user-generated content represents a gold mine for organizations to develop. a significantly deeper understanding of their customers using AI-based sentiment analysis tools. Using NLP techniques to analyze a particular person's online content, this tool can develop a very specific understanding of that person's character, as shown in Figure 24.
For example, in the wealth management industry, IBM Watson Investment Advisor can create relationships between a client's personality, life situation and the vast ocean of financial market data. These inputs can be matched against different investment alternatives to recommend an optimal personalized wealth management strategy.
Enterprise AI: Working Smarter & Harder on Behalf of Professionals
Expert Assist: This refers to AI-based systems that enable knowledge workers to retrieve and produce information in a highly efficient manner. In 2016, the American newspaper The Washington Post deployed Heliograf, a homegrown AI-based solution that could generate short reports based on stories, facts and data provided by human reporters. Input Management: This concerns the automatic (pre)processing of incoming mail, e-mails, invoices, spreadsheets, presentations, PDFs and other documents using AI.
VKB, an insurance company in southern Germany, has implemented an AI-powered input management tool using IBM Watson to identify the subjects and sentiments from unstructured text in incoming emails and letters. Researchers at IBM Watson estimated that there were 17 trillion PDFs in all enterprises in September 2017.24 AI helps to understand different data formats and enables companies to analyze relevant data.
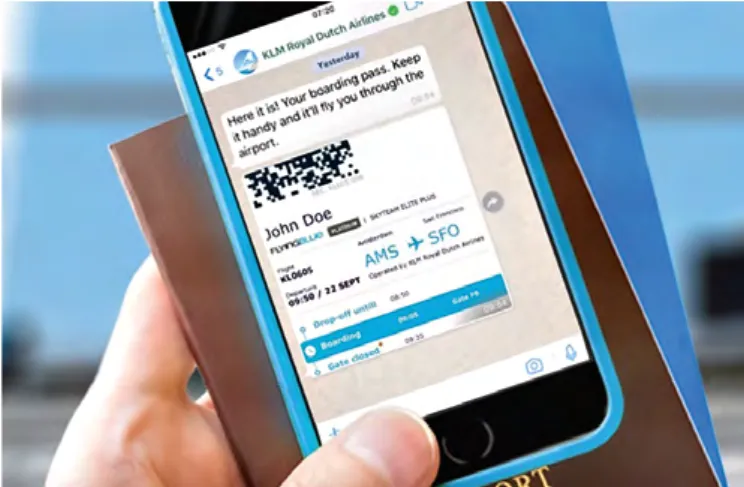
AI in Retail: Personalized Online Experiences & Self-Learning, Replenishing Spaces
Content Discovery: This refers to the automatic analysis of unstructured data from emails, PDFs, images, audio and video, enabled by the evolution of big data analytics tools and using AI. Most companies have a lot of this unstructured data; these typically represent 80% of all business data and are usually not analyzed. The Frauenhofer Society, a leading technical research institute in Germany, uses this approach to identify emerging scientific trends and assign researchers accordingly.
American home improvement retailer Lowe's uses a customer service robot called OSHbot, developed in conjunction with Fellow Robots, to help customers find information about a specific product and then guide the customer to where they can find that product. OSHbot can perform real-time inventory level checks and, as a mobile telepresence unit, can connect employees between stores.
Autonomous Transportation: AI Under the Hood
Here, deep learning algorithms, natural language processing and computer vision take care of both recognizing the item, down to perhaps a single nail or bolt, and understanding the customer's request. By predicting defects and breakdowns using AI, GE has been able to reduce unplanned shop floor downtime by 20% and significantly improve product quality, throughput and yield.29. In the agricultural sector, John Deere is looking for ways to feed a world populated by 10 billion people with access to only a finite amount of arable land.
They can even communicate with Watson via voice commands, utilizing the system's natural language processing capabilities, so they can remain hands-free while working on the shop floor. Thanks to the falling cost of components, the increasing performance of deep learning algorithms and the growing collective knowledge of the transport sector in the field of autonomous driving, assistive technology is developing rapidly.
Engineering & Manufacturing: AI Shapes the Physical World
The agricultural machinery company is using IBM Watson and a smart manufacturing platform to deliver AI-powered assembly and maintenance at its largest manufacturing plant in Mannheim, Germany. The system is connected to an intelligent order management system, so once the problem is understood and the required parts are identified from the image, the correct parts order can be automatically placed. Convenience, cost reduction and increased efficiency in the form of lower emissions and fewer accidents are the primary drivers for autonomous vehicle use.
However, full implementation – in other words, a driverless vehicle in the legal sense – will require significant regulatory changes in any country, and this will take some time. As explained in the previous chapters, Artificial Intelligence (AI) is increasingly present in our personal lives and is rapidly being adopted by businesses to increase efficiency and create new value.
Back Office AI
The system uses natural language processing to classify potential contract clauses, policy-relevant sections, and signature parts. American startup CircleBack has developed an AI engine to help manage contact information that continuously processes billions of data points to determine whether contact information is accurate and up-to-date.34 Additionally, as discussed in Chapter 2, AI tools can , trained in input management, use natural language processing to perform some pre-processing of customer address information to ensure completeness, correctness and compliance with global and regional address formats. The solution is AI for customs brokerage; an enterprise AI platform such as IBM Watson can be trained with legislative materials, regulatory documents, SME customs brokerage knowledge, and customer and industry manuals to learn how to automate customs declarations.
Using natural language processing and deep learning's self-learning capabilities, a customs broker's AI could input customs documents in a myriad of formats, extract relevant information using its accumulated knowledge, and submit an automatic declaration. When the AI-based system encounters an exceptional case, a human customs broker can review the declaration.

Predictive Logistics: The Next Operational Paradigm
DHL's Global Trade Barometer is a unique tool for early indication of the current state and future development of world trade. The DHL Resilience360 platform is a cloud-based supply chain risk management solution tailored to the needs of global logistics operators. Supplier issues, from material shortages to poor labor practices and even legal investigations, can cause critical supply chain disruptions.
This in turn allows supply chain managers shown in Figure 40 to take corrective action earlier and avoid disruption. These images provide rich sources of data for the development of advanced mapping tools to increase the accuracy of pick-up, navigation and departure between drivers and their riders.
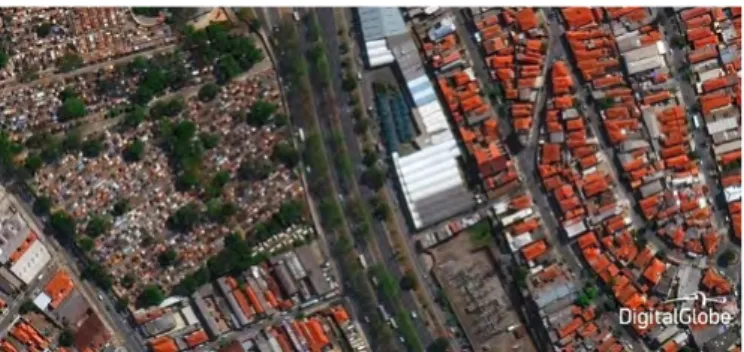
Seeing, Speaking & Thinking Logistics Assets
AI-Powered Visual Inspection is another area with high potential for AI in the logistics environment. French startup Qopius develops computer vision-based AI to measure shelf performance, track products and improve retail store execution. In inventory management, similar use of computer vision AI offers potential for real-time inventory management at the individual piece and SKU level.
Before the parallel advancement of AI and speech recognition technologies, voice-activated tools in the supply chain were static; they were limited to keywords and audible menus, and operated with fixed commands. A more practical application of autonomy in the last mile comes in the form of autonomous unmanned ground vehicles (UGVs) that operate fully autonomously or in collaboration with a delivery person.
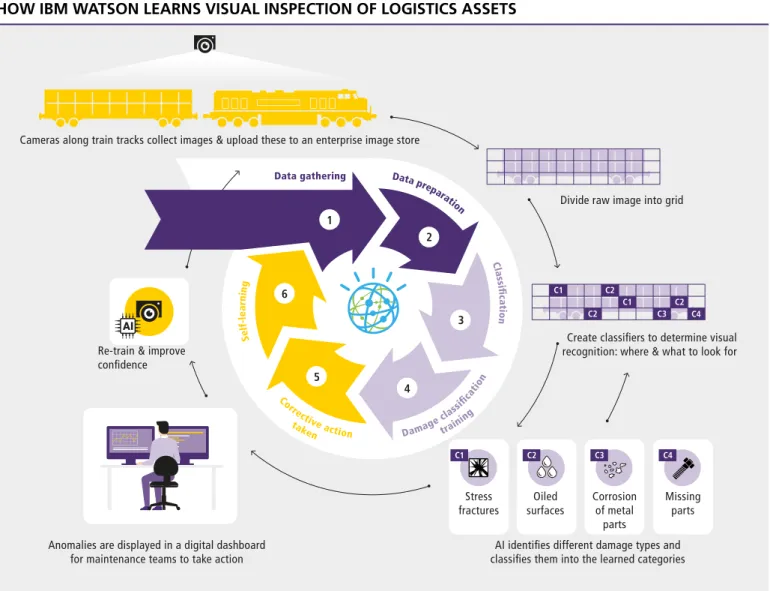
AI-Powered Customer Experience
Embedded conversational AI helps improve human-robot interaction; if a person blocks Robby's way, a soft voice politely asks "excuse me" and will even say. Anticipatory Logistics takes the AI-powered logistics customer experience to the next level, delivering goods to customers before they've even ordered them or realized they needed them. Anticipatory logistics seeks to leverage AI's capabilities to analyze and draw predictions from vast amounts of data such as browsing behavior, purchase history and demographic norms, as well as seemingly unrelated data sources such as weather data, social media chats and news reports to predict what customers will buy.
By exposing these data sources to AI analytics, companies can effectively forecast demand and reduce delivery times by moving inventory closer to customer locations and allocating resources and capacity to meet previously unforeseen demand. In some cases, unpurchased inventory would even need to be constantly on the move to allow immediate delivery of an order placed while the goods are in motion.
Getting Started with AI in Your Supply Chain
Tech giants around the world are moving towards AI-first product strategies; In: ishir.com.
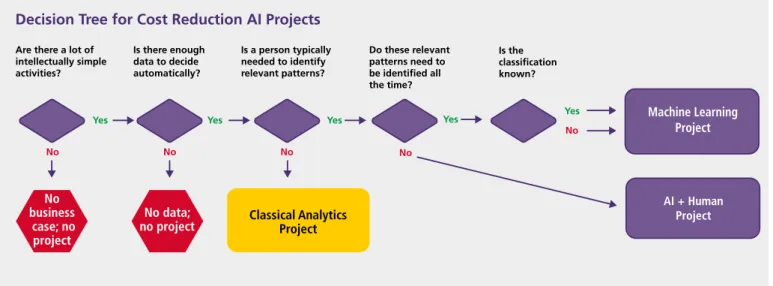