Embracing the experience of formulating creative questions that test creative abilities and evaluate students' creative responses. To systematically identify features of creative questions that instigate creative responses among students of human-centered design approach.
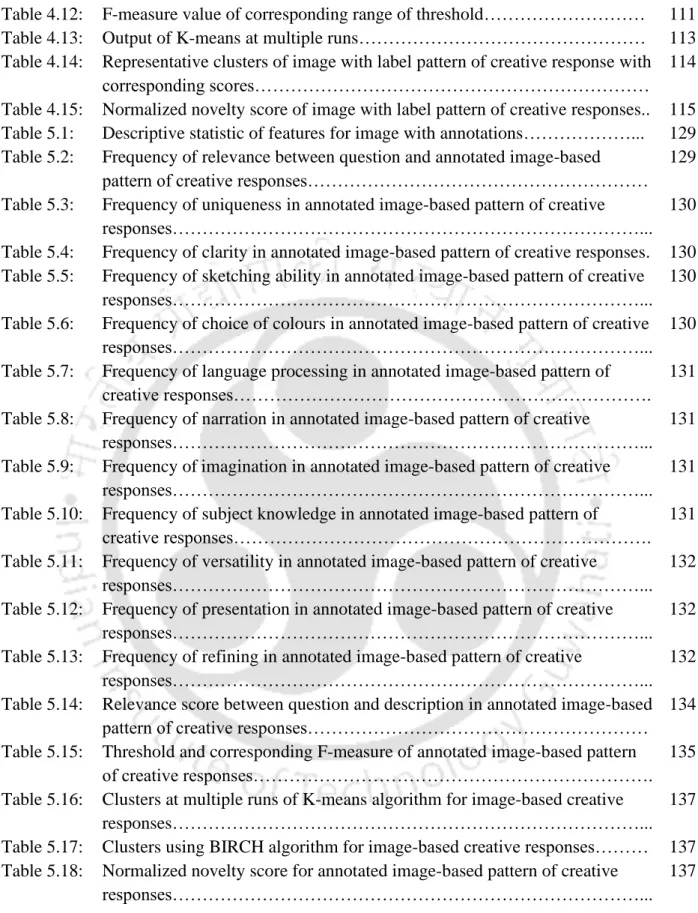
Introduction 1
Overview of Design entrance examinations in India
The objective part consists of questions related to numerical, logical, etc., whereas the subjective part possesses questions related to sketching, shape sensitivity, visual sensitivity, problem identification, design thinking, etc. Question patterns in these nationalized design exams are structured into two categories, namely, objective and subjective question patterns .
Questions instigating creative responses…
On the other hand, subjective questions accept open-ended answers that depend on students' unique ideas. Open solutions are not pre-defined and are currently evaluated based on the novelty of an answer by domain-specific experts.
Creative aptitude evaluation in Design education
Furthermore, a response that has never been experienced before is considered complete novelty (Barto et al., 2013). Rare responses compared among cohort of students in an exam are considered to have relatively higher novelty (Omari et al., 2016).
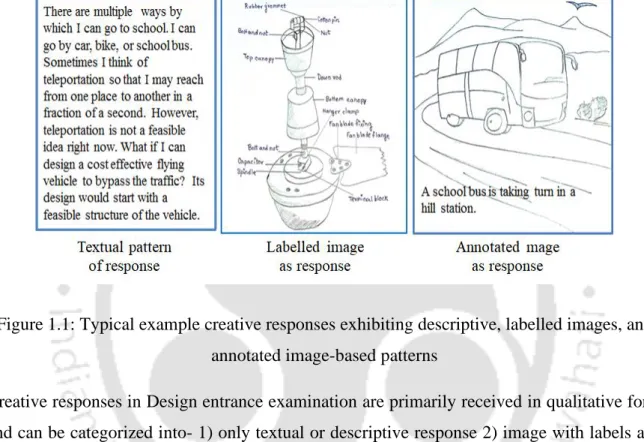
Role of Design pedagogues in assessing creative aptitude
In such situations, experts can become frustrated and fatigued by repetitive monotonous tasks performed on a large scale. Different raters may have different frames of reference for judging the answers, resulting in inconsistency in the evaluation process. Differences of opinion between different assessors arising from individual frames of reference can lead to major inconsistencies in the evaluation process.
State of the art review
- Features of questions triggering creative responses
- Novelty in descriptive or textual content
- Novelty in images or sketches
- Stress associated with pedagogy-related tasks
It did not necessarily focus on the students' ability to generate creative responses to the problem provided in the question (Kurdi et al., 2020). Performance metrics showed that this model outperformed other state-of-the-art approaches (Potamias et al., 2020).
Research gap
There is a dearth of literature that addresses the stress of educators in assessing creative skills in design education. The result of the literature review highlighted that there is a lack of studies that have ever investigated the ways in which educators design creative questions for assessing students' creative abilities.
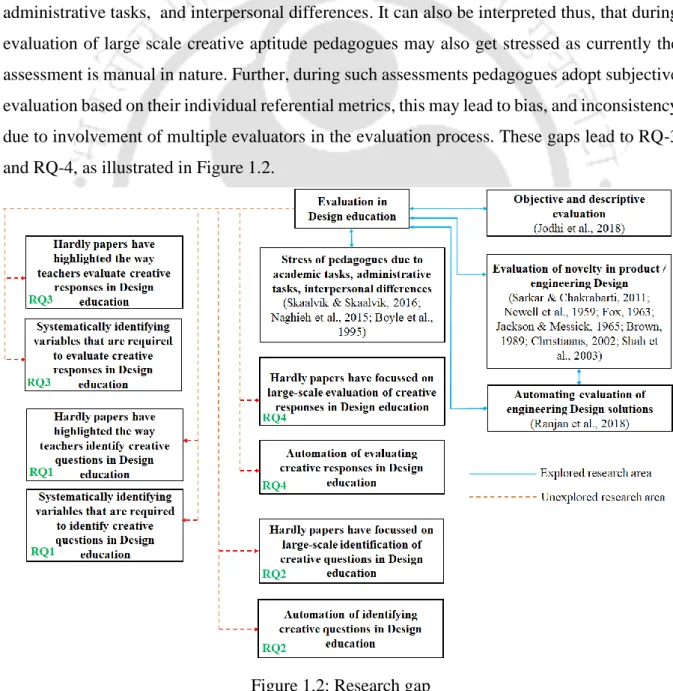
Motivation of the research study
Research questions (RQ)
Aim
Objectives
Focus of research
Expected outcome(s)
Structure of the thesis
RQ3: What factors do design teachers consider when evaluating students' creative responses when evaluating response novelty. Identification of factors and automated evaluation of annotated image and creative response pattern based on annotation].
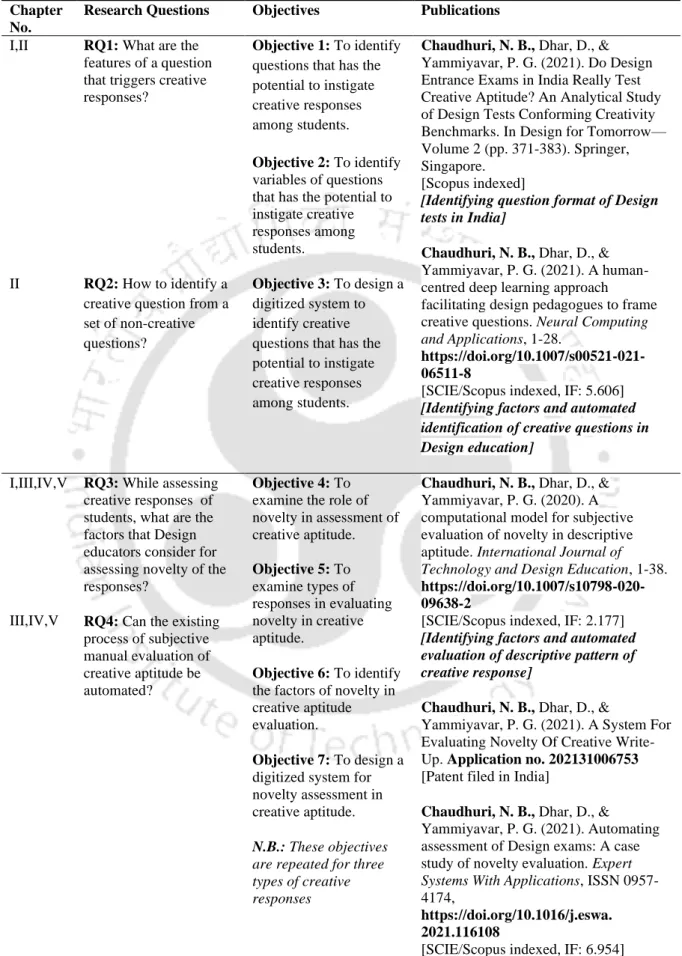
Summary of chapters
Furthermore, the design of the computational model to automatically evaluate labeled image-based creative response is shown. Furthermore, the design of the computational model to automatically evaluate annotated image-based creative response is illustrated.
Introduction
This study is not focused on identifying creative question features through peer review techniques, observations, or individual subjective assessment. The characteristics of creative questions (f1, f2,…, fn) were taken from educators associated with design education with experience in formulating creative questions.

Method
- Questionnaire preparation and interview
- Mixed-method analysis
- Feature extraction
- Data preparation
- Identifying creative questions
The 'open questions' feature was considered the basic criteria for a query to be registered as creative by experts. Kaggle's Google-quest (Google QUEST Q&A Labeling | Kaggle, 2020) data has 31 features, from which "question identification" refers to a specific question, and nine features associated with solutions were excluded.
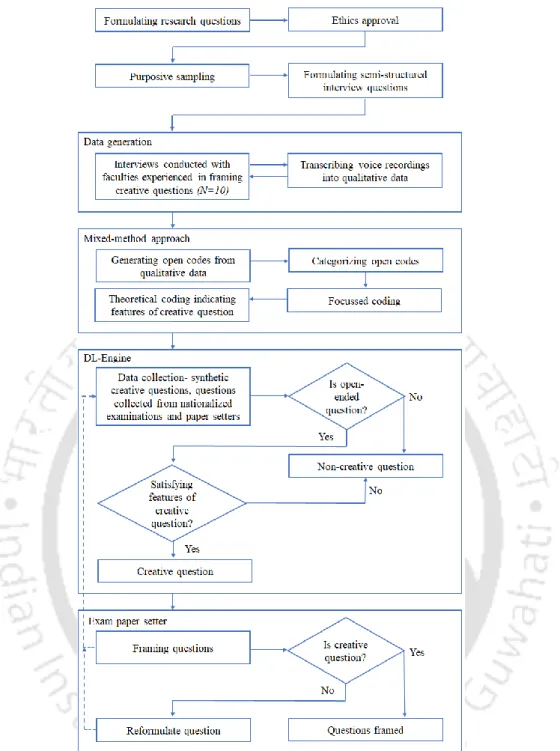
Results and discussion
- Mixed-method research results
- Machine learning research outcomes
- Optimizing decision-making of examination paper setters
- Performance evaluation with baselines
- Validation of the proposed model
I feel that there is no specific process that I can tell you.”, “Questioner must himself be a very good teacher and a creative person. However, this study focused on a systematic approach using a qualitative study to acquire the features of creative questions. The limitation of the current methodology is that it largely focuses on identifying creative issues specifically in design education.

Conclusion
Therefore, this chapter attempts to find the innovation evaluation parameters in the descriptive model of creative responses and further support educators by automating the innovation evaluation process using a proposed computational design model. The survey resulted in characteristics that closely resemble human evaluation strategies for evaluating novelty from the descriptive model of creative responses. Human-centered design approach to identify parameters of evaluation of novelty in creative descriptive answers of entrance exams.
Introduction
A scientific study is planned, which intends to conduct a survey to determine the characteristics of innovation evaluation in creative ability in the design fraternity. To answer this research question, a factor-based model of novelty evaluation is proposed, which attempts to automate the evaluation process. Finally, the outcome of the model is validated by comparing the results with the gold standard data.
Method
- Questionnaire preparation and survey plan for identifying
Coherence between responses was identified by Bidirectional Encoder Representations from Transformers (BERT), which predicts the probability that the next sentence is the next sentence. The first value, when passed through a normalization function, gave the probability that the next sentence is the next sentence. The second value, when passed through a normalization function, gave the probability that the next sentence was not the next sentence.
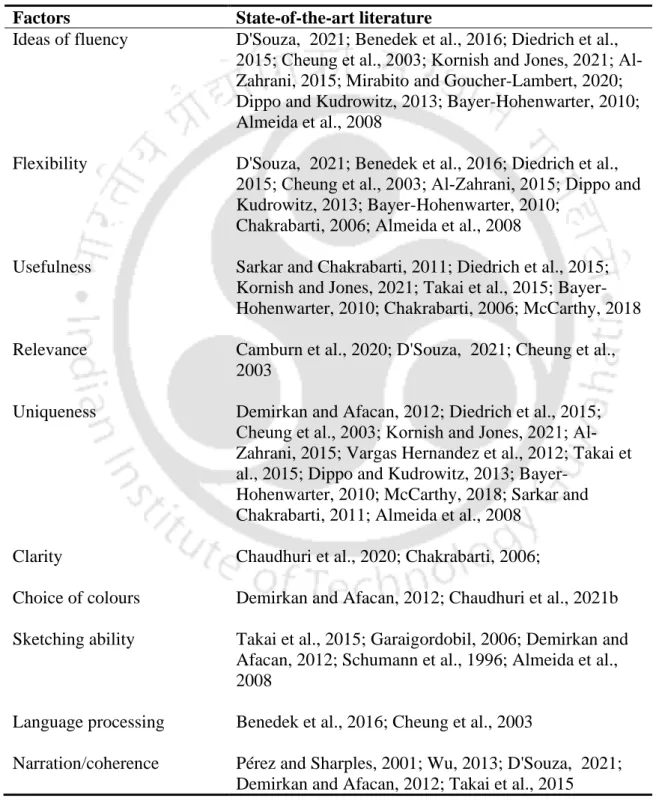
Results and discussion
- Data collection and pre-processing
- Language processing
- Relevance between question and creative responses
- Coherence in a response
- Consequences of summarization and without summarization
- Relative uniqueness of a creative response
- Novelty score and model validation
1 + 𝑇𝐺 (3.1) Where N is the number of grammatical errors and TG is the threshold value for that category given in equation 3.2. 1+𝑇𝑆 (3.4) Where N is the number of spelling errors and TS is the threshold for that category given by equation 3.5. 1 + 𝑇𝑁 (3.8) Where N is the number of narration pauses and TN is the threshold for that category given by equation 3.9.
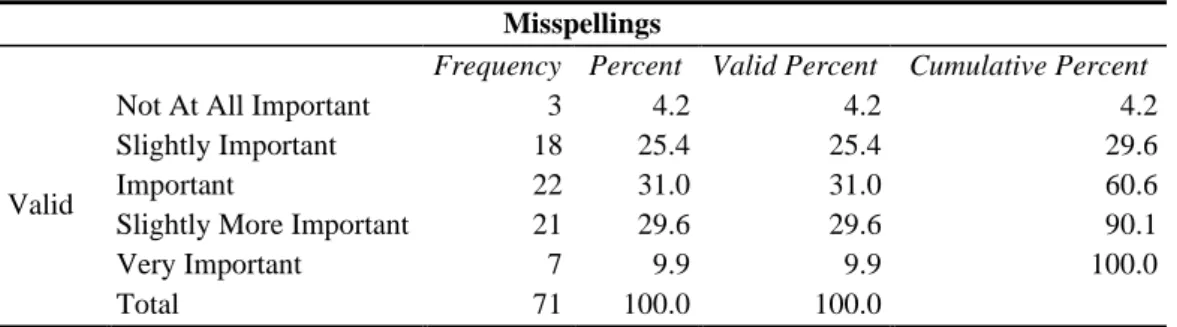
Conclusion
To mitigate these challenges, a computational design model is proposed for the automatic evaluation of novelty in an image-based labeled pattern of creative responses. A human-centered design approach for identifying novelty assessment parameters in an annotated image-based pattern of creative responses. Proposing a computational design model for novelty evaluation in a labeled image-based creative response pattern.
Introduction
These features were then used to conceptualize a model that evaluates and scores the novelty of creative responses. Initially, the proposed model aimed to identify whether a labeled image-based creative response pattern was relevant to a question. Groups that possessed fewer images were considered relatively more unique by the proposed model.
Method
- Survey plan and participants
- Questionnaire and parameters of evaluation
- Detailed architecture for evaluating image with labels
- Dataset for image with labels
- Pre-processing data of image with labels
- Prediction of image with labels
- Thresholding creative responses of type image with labels
- Clustering and evaluation of novelty of image with label pattern of
- Survey site
- Descriptive statistic of features of image with labels identified by
- Relevance score between question and image with label pattern of
The simplicity of an image with the creative answer tag template refers to a layout that is easy to perceive. First, the image corresponding to the label pattern of creative responses was clustered, which grouped semantically similar responses together. Initially, the K-means algorithm was used for clustering high dimensional image vectors with creative response label pattern.
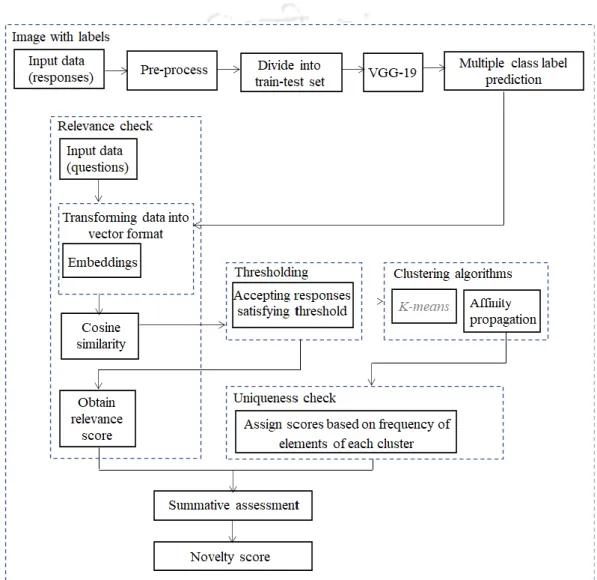
Conclusion
This model is developed using mixed methods research, where the novelty rating features of creative response patterns based on labeled images are investigated by conducting a survey. A Human-Centered Design Approach for Identifying Novelty Evaluation Parameters in an Annotated Image-Based Creative Response Pattern. To propose a computational design model for novelty evaluation in a sample of creative responses based on annotated images.
Introduction
To address these kinds of exceptions, a computational design model is proposed that attempts to digitize the evaluation of novelty in annotated image-based patterns of creative responses. This activates two activities, namely identifying traits to evaluate novelty in annotated image-based patterns of creative responses that demonstrate creative aptitude and proposing a model that attempts to digitize the evaluation process based on these traits. The proposed model was initially intended to determine whether an annotated image-based pattern of creative answers is relevant to a question or not.
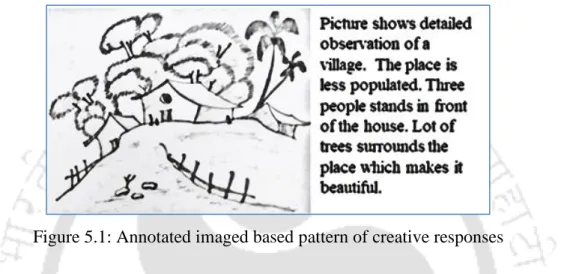
Method
- Survey plan and participants
- Questionnaire and parameters of evaluation
- Detailed architecture for evaluating image with annotations
- Dataset for image with annotations
- Pre-processing of data for image with annotations
- Prediction of image with annotations
- Relevance between question and annotated image-based pattern of
- Clustering and evaluation of novelty of annotated image-based
- Survey site
- Descriptive statistic of features of image with annotations identified
- Relevance score between question and annotated image-based
- Validation of the proposed model
Multi-label object recognition was essential for identifying multiple elements in annotated image-based patterns of creative responses. Imaginatively, any annotated image-based pattern of creative responses represents creative and divergent ideas. The proposed models for evaluating novelty from an annotated image-based pattern of creative responses required validation.

Conclusion
Experimental results and research findings from "2. chapters" to "5. chapters" are briefly explained in the present chapter with the key findings of the thesis. The purpose of these proposals is to prepare the design education community, specifically design educators, to embrace changes in existing ways of framing creative questions and evaluating their answers. In this chapter, the novelties in the contribution of this research are also indicated from the point of view of the knowledge base, methods, design and design education.
Discussion
- Key findings of the thesis
A computational model was designed and developed to evaluate novelty in labeled image-based patterns of creative responses. A computational model was designed and developed to evaluate novelty in annotated image-based patterns of creative responses. The performance metrics of the proposed models for evaluating descriptive, labeled image-based, and annotated image-based patterns of creative responses were measured.
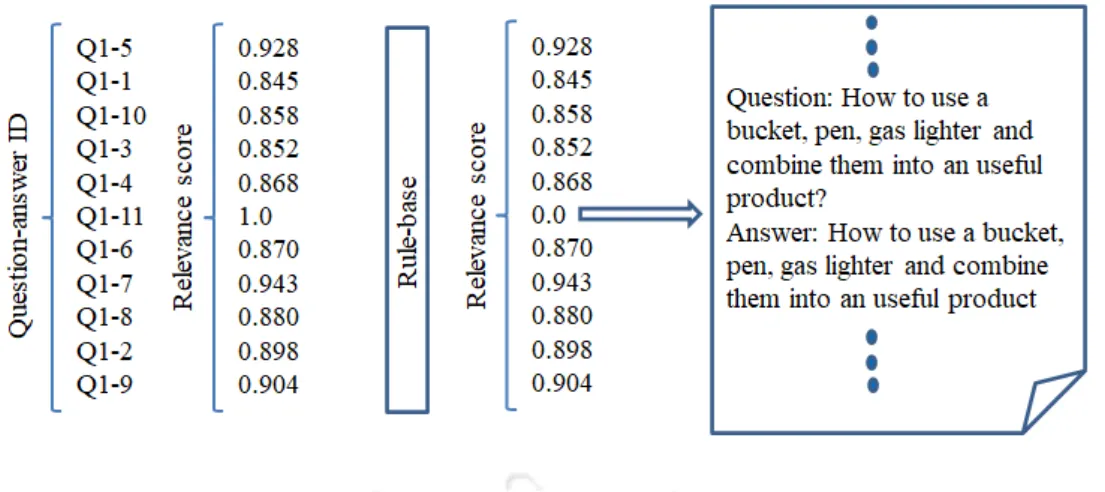
Fulfillment of objectives
These features support finding relevance between a question and a sample of creative answers based on an annotated image and the uniqueness of the answer. Two subfactors of novelty were identified in the mass review for the image-based labeled sample of creative responses, namely question-answer relevance and relative uniqueness of the answer. Three sub-factors of novelty were identified in the mass review for the sample of creative responses based on the commented image, namely linguistic processing, relevance between the question and their responses, and relative uniqueness of the response.
Validation of the models
A computational design model was proposed that assessed novelty in the creative response descriptive model that illustrates creative ability. A computational design model was proposed that assessed novelty in the image-based labeled model of creative responses that exemplified creative ability. Validation of the model for evaluating the annotated image-based creative response model A computational design model was proposed that evaluated the novelty in the annotated image-based model of creative responses illustrating creative ability.
Implications
- Implication from the perspective of design process
- Implication from the perspective of human-centred approach
The human-centered approach is mainly related to fields such as ergonomics, human-computer interaction and artificial intelligence. Moreover, a particular design of a model was accepted depending on the performance of the proposed model. The design of the model was confirmed depending on its closeness to the human-based evaluation results.

Recommendations
The limit was variable and could change depending on the type of exam, the level of the exam, the severity or leniency of the pedagogue. This research paper recommends the use of twenty-two variables to identify questions that elicit creative responses, five variables to assess novelty in descriptive creative responses, two variables to assess novelty in labeled pictorial creative responses, and three variables to assess novelty in labeled images. based on creative responses. The threshold for each parameter of the model is flexible, so it is recommended to change the threshold values according to the type of exams, the difficulty of the exams, the strictness or leniency of the pedagogues.
Novelties in contribution of the present research
- Contribution to knowledge-base
- Contribution toward methods
- Contribution to Design and Design education
Reference questionnaire to identify creative questions and assess innovation in creative answers in the Design entrance exam. An important contribution from a methods perspective is the proposed architectures to identify creative questions and evaluate innovations in creative answers. Therefore, this architecture can act as a scaffold to identify creative questions and evaluate the novelty of creative answers for a large-scale design acceptance exam.
Limitation and future scope
The results of this thesis are significant, as they will support increased confidence in subjective evaluation in design education. This thesis contributes to the assessment system design process as it supports the systematic identification of features in the evaluation of creative abilities and the identification of creative questions that instigate creative responses. The design of the computational models will support a consistent evaluation and identification of creative questions.
Conclusion
Proceedings of the 39th ACM SIGIR International Conference on Research and Development in Information Retrieval, 215–224. Proceedings of the 42nd ACM SIGIR International Conference on Research and Development in Information Retrieval, 1113–1116. Analysis of mean absolute error (MAE) and root mean square error (RMSE) in rounding model estimation.