48 3.13 Mean height distributions of the CHts1 child test group signals after. ASR performances for the CHts1 child test set on models trained on the ADtr adult speech dataset within the baseline, ∆ and ∆∆ feature streams.
Overview of Automatic Speech Recognition
PEAKS is an automated speech intelligibility assessment system that can be accessed over the Internet [23]. However, the success of ASR technology lies in the development of a robust speech recognition system that can perform well regardless of the usual differences in training and testing conditions.
Challenges in Children’s Speech Recognition
Acoustic Correlates of Children’s Speech
Glottal flow parameters, which control the shape of the glottal pulse, affect the overall long-term shape (spectral skew) of the speech power spectrum [41, 42]. The average Euclidean distance between the cepstral coefficients of the first and second halves of vowels for 5-year-old children is 20%.
Linguistic Correlates of Children’s Speech
Increased spectral and temporal variabilities within speakers cause greater overlap of phonemic classes making the pattern classification problem even more difficult. So, children's ability to use language efficiently to convey the message improves as they age.
Performances of ASR Systems for Children’s Speech
They compared human and machine recognition performance on the same speech data of adults and children. Human recognition performance for children's speech has been shown to exhibit similar age effects to those observed for automatic systems.
Review of Approaches used for Children’s ASR
Acoustic Mismatch
- Feature Domain Approaches
- Signal Domain Approaches
- Model Domain Approaches
In [6], Narayanan and Potamianos reported 45% improvement in the WER for children's ASR on adults' speech-trained models by VTLN on a digit recognition task. Constrained MLLR speaker normalization (CMLSN) and speaker adaptive training (SAT) have also been studied for improving children's ASR on the adults' speech-trained models [30,69].
Linguistic Mismatch
However, most of the aforementioned studies pointed out the lack of children's acoustic data and tools to estimate speech recognition parameters compared to the plethora of existing tools for adult speech recognition. The availability of larger amounts of children's speech data allowed the re-examination of age-dependent and speaker-adaptive acoustic modeling, in the context of the continuous speech recognition tasks of children with average and large vocabularies.
Motivation of the Thesis
They compared the effectiveness of linear prediction cepstral features and MFCC features for recognizing children's speech on trained adult speech models in a digit recognition task related to telephone speech in [48].
Objectives of the Thesis
Organization of the Thesis
In Chapter 7, MFCC feature truncation is explored for pitch discrepancy reduction for recognizing child speech on trained adult speech models. The efficiency of the proposed algorithm is also explored in combination with existing speaker normalization and model fitting techniques for children's ASR on trained adult speech models.
Speech Corpora
In this chapter, the speech corpora used in this thesis are described for performing several ASR experiments on both the connected digit recognition task and the continuous speech recognition task. The details about all these speech corpora used in this thesis are given in Table 2.1.
Speech Recognition Systems
Connected Digit Recognition
Adult speech recognition performance on trained child speech models is evaluated using the same adult speech dataset 'ADts'. The baseline recognition performance (in WER) for the ADts and CHts1 test sets in the trained adult speech digit recognizer is 0.43% and 11.37%, respectively.
Continuous Speech Recognition
The baseline recognition performance (in WER) for CAMts and PFts test sets on the adult speech-trained continuous speech recognizer is 9.92% and 56.34%, respectively. This thesis focuses on children's speech recognition performance on adults' speech-trained models, i.e., under mismatch conditions.
Methods for Transformation of Acoustic Correlates of Speech
Signal Domain Method: PSTS
- Transformation of Pitch and Signal Duration
- Transformation of Glottal Flow Parameters
To perform speech-synchronous LP analysis, the pitch signatures of the speech signals are extracted. In the unaltered regions of the speech signals, the pitch cues are kept 5 ms at an equal distance.
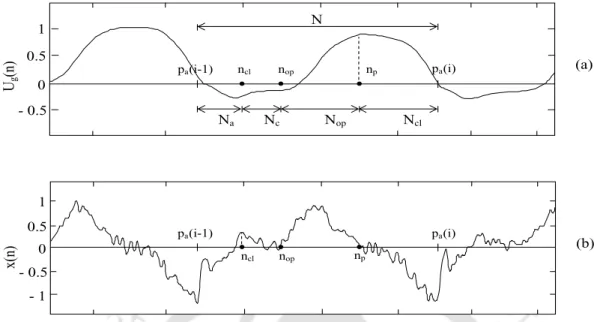
Feature Domain Method: VTLN
The return coefficient is related to the return phase duration and determines the cutoff frequency of the spectral tilt. The return coefficient can be increased or decreased by a time-scale expansion or compression of the return phase.
Model Adaptation Techniques
MLLR
- MLLR-MEAN
- MLLR-COV
In this work, the effect of fitting the mean and variance parameters of the models is studied separately. The fitting method, in which linear transformations are applied only to the variances of the models, is called "MLLR-COV" in this thesis.
CMLLR
When a single transformation is used to fit all Gaussian densities in the recognition system, the CMLLR fit can be implemented by transforming the acoustic observations [71]. Since multiple CMLLR transformations can be used, it is important to include the Jacobian in the likelihood calculation.
Summary
Effect of Various Acoustic Sources of Mismatch on MFCC Features & ASR Models
Pitch
Speaking Rate
Glottal Flow Parameters
Formant Frequencies
Relative Significance of Various Acoustic Sources of Mismatch for Children’s ASR
Connected Digit Recognition Task
- Pitch
- Speaking Rate
- Glottal Flow Parameters
- Formant Frequencies
The recognition performance of the children's test set CHts1 with and without explicit speech rate normalization is given in Table 3.4. The ASR capabilities of the CHts1 children's test set with and without VTLN are given in Table 3.4.

Continuous Speech Recognition Task
Combining VTLN and Explicit Acoustic Normalization with Model Adaptation
It is noted that the average of the speech rate distribution of the adults' training set ADtr is 1.2 times higher than that of the children's test set CHts1. This verifies the reduction in the previously hypothesized mismatch in the duration modeling of the children's test set with respect to the adults' speech-trained models.
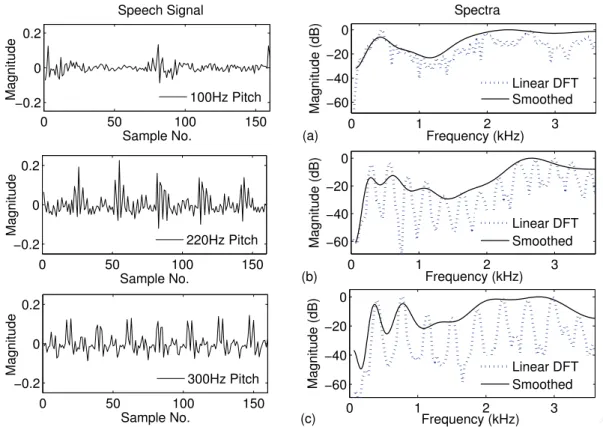
Summary
No significant effect of variations in glottal flow parameters is observed on children's ASR performance on trained adult speech models. Sustained and significant improvement was achieved in children's ASR performance in both related number recognition and continuous speech recognition tasks after clear normalization of children's speech pitch.
Effect of Uniform and Non-Uniform Filterbank on Pitch Harmonicity
Uniform Filterbank based Spectral Analysis
However, pitch harmonics are clearly discernible in the uniform spectrum based on the high vowel filter bank. This results in a smoothing of the pitch harmonics in the linear DFT spectrum of the low-tone vowel frame, while they are preserved in the case of the high-tone vowel frame.
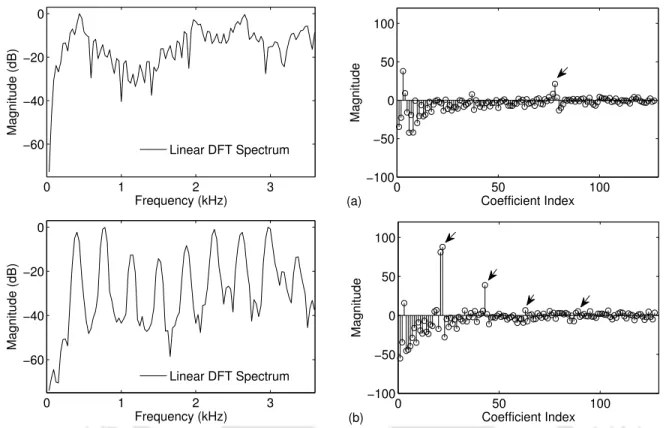
Non-Uniform Filterbank based Spectral Analysis
The bandwidths of the filters in the Mel filter bank increase with increasing center frequencies of the filters. This verifies that the pitch-dependent distortions appear only in the Mel spectral envelope for high pitch signals due to the insufficient smoothing of the pitch harmonics by the Mel filter bank.
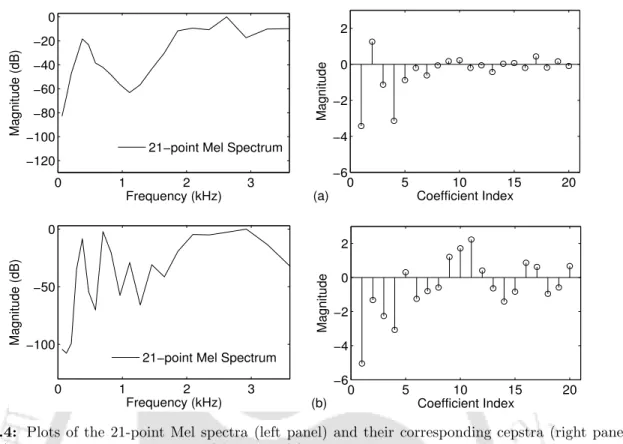
Effect of Pitch-dependent Distortions on MFCCs
As the pitch frequency increases, the dynamic range of the higher-order MFCCs increases relatively more than that of the lower-order MFCCs. This is attributed to the increase in the variances of the higher order coefficients of 13-D MFCC (C0−C12) with increase in the pitch of the signals, as noted in Section 3.2.1.
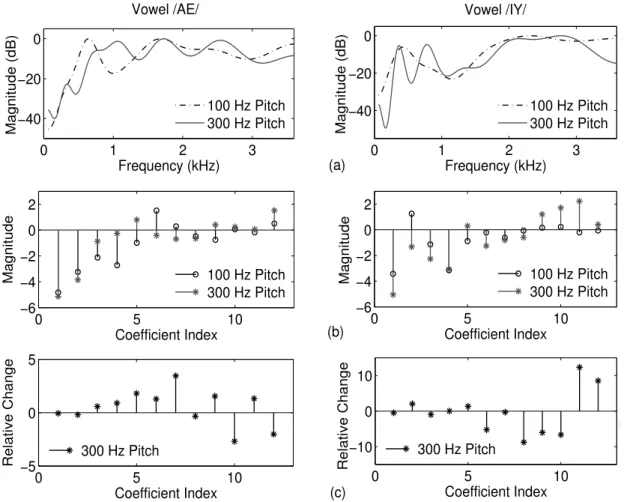
Summary
Regardless of the acoustic characteristics of a speech signal, MFCC functions are used to parameterize the speech signals of both adults and children. As a result, there could be significant degradation in children's ASR performance on adult speech-trained models using MFCC features.
Efficacy of PLPCC Features for Children’s ASR
Effect of Pitch on PLPCC Features
The mean and variance of the squared MD of the signals of different pitch groups for the /AE/ and /IY/ vowels corresponding to the PLPCC features (C1−C12) are given in Table 5.1. However, the mean and variance of the squared MD of the PLPCC features of the loud set signals are much lower than those of the MFCC features of those signals for both vowels.

Children’s Speech Recognition using PLPCC Features
This range of transformed height values is chosen taking into account the step range of the adult training sets and the child test sets. The Fo <250 Hz sound group matches the sound range of the adult training speech data.
Children’s ASR using PMVDR Features
For child speech recognition, the baseline performances of the CHts1 child test set using standard PMVDR features and MFCC features (for ease of comparison) are given in Table 5.3, along with a breakdown for different pitch groups. The speech recognition performances of the child test set CHts1 after explicit pitch normalization for PMVDR features and MFCC features (for ease of comparison) are also given in Table 5.3 along with a breakdown for different pitch groups.
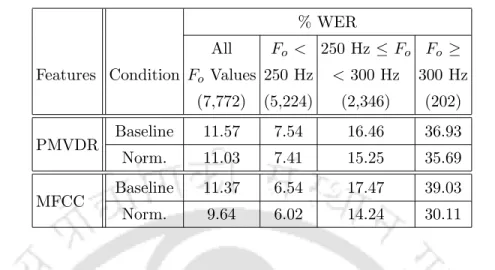
Summary
It is noted that significant improvement in children's ASR performance is achieved with explicit ML-based pitch normalization of children's speech using MFCC features. Due to the constant-Q type Mel filterbank used in MFCC function calculation, there is no harmonicity in the pitch-dependent distortions appearing in the Mel spectrum.
Mel Filterbank Modification for Pitch Normalization
Implicit Modification of Filter Bandwidths
The ten different values chosen for the number of filters in the filter bank for MFCC include the calculation of child speech from 12 to 21 in steps of 1. Changing the number of filters in the Mel filter bank results in an uneven modification.

Selective Modification of Filter Bandwidths
On connected digit recognition task, the best ASR performances are obtained for the signals of the children's test set CHts1 with average pitch values of less than 250 Hz using constant bandwidth of 250 Hz for the first 3 filters in the Mel filter bank. On continuous speech recognition task, the best recognition performances for the signals from the children's test set PFts with average pitch values of less than 250 Hz are obtained using constant bandwidth of 250 Hz for the first 11 filters in the Mel filter bank.
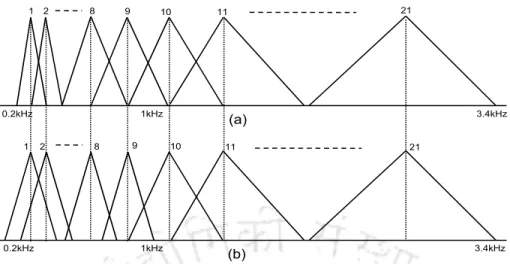
Proposed Pitch Normalization Algorithm
Based on these, we further investigated the effectiveness of the proposed change in the Mel filterbank for pitch normalization for children's ASR on speech-trained child models. It should be noted that the improvement in ASR performance in children on matching models with the proposed algorithm is greater than that reported by [63].
Combining Proposed Algorithm with VTLN and CMLLR
It should also be noted that the relative gain achieved by VTLN when performed in conjunction with the proposed filterbank-based pitch normalization is comparable to that achieved by VTLN in the standard case. The relative gain obtained with CMLLR when performed in conjunction with the proposed filterbank-based pitch normalization is also comparable to that obtained with CMLLR in the standard case.
Summary
Based on this, this chapter investigates the trimming of MFCC functions for children's ASR on speech-trained adult models. Next, the role of truncation of MFCC features in reducing the pitch mismatch between adult and child speech is investigated.
Truncation of MFCC Features for Children’s ASR
Thus, greater degree of cepstra truncation of standard base MFCC features helps children's speech recognition on the adults' speech-trained models. It should be noted that some coefficients of the standard 39-D MFCC functions appear to have no significant role in children's ASR on adult speech-trained models.
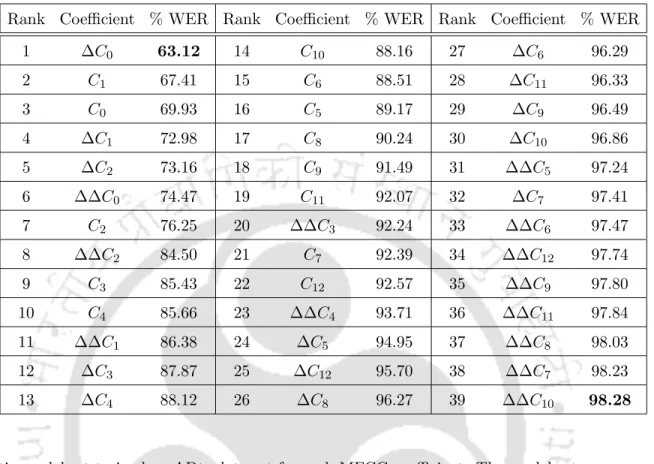
Role of MFCC Feature Truncation in Pitch Mismatch Reduction
The table also shows the ratio of the variances of the squared MD of the feature vectors for 'low' and 'high'. This indicates that with the increase in truncation of MFCC features, the feature vectors of high-pitch group signals come closer to the distribution of MFCC features of the 75–100 Hz pitch group signals to an extent similar to that of the low-pitch group, but more so when higher order MFCC is truncated by reducing pitch mismatch.
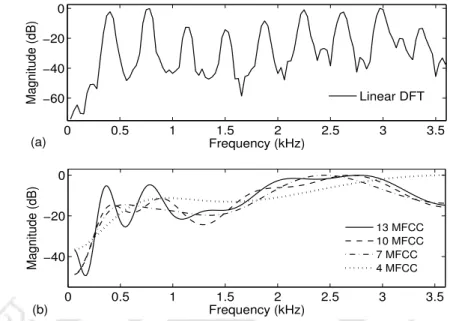
Adaptive MFCC Feature Truncation for Pitch Mismatch Reduction
Correlation between MFCC Feature Truncation and VTLN Warp Factor
The VTLN distortion factor-wise recognition performance of the child test sets CHts1 and PFts for different dimensions of the truncated test features on corresponding adult speech-trained models with corresponding feature dimensions are given in Table 7.6 and Table 7.7, respectively. The corresponding ASR performance of the adult speech test sets ADts and CAMts with varying MFCC feature truncation, along with their VTLN warp factor-wise performance, are given in Table 7.8 and Table 7.9, respectively.
Proposed Algorithm for Adults’ Speech Trained ASR Models
The flowchart of the algorithm proposed for ASR on adult speech-trained models is shown in Figure 7.3. When recognizing the adult test speech data, a slight, insignificant reduction in ASR performance is observed compared to baseline on the adult speech-trained models.
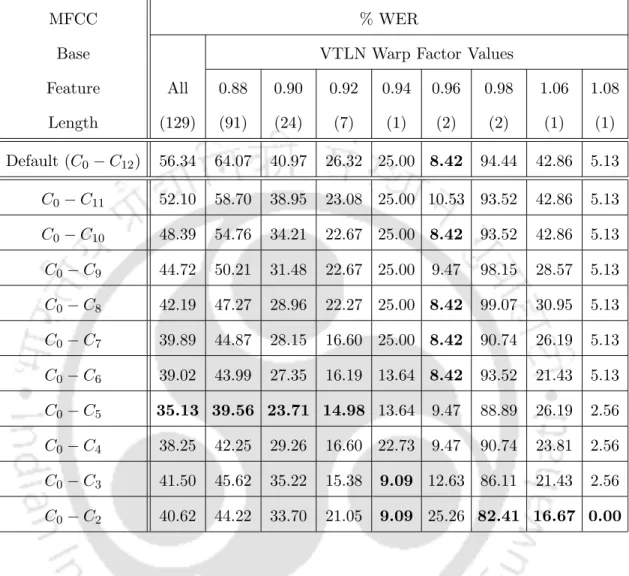
Proposed Algorithm for Children’s Speech Trained ASR Models
This statement can be further substantiated by comparing the mean Bhattacharyya distance (BD) [138] between telephone classes for adult and children's speech-trained telephone models. The average BD for the adults and the children's speech-trained phone models is shown in Figure 7.5.
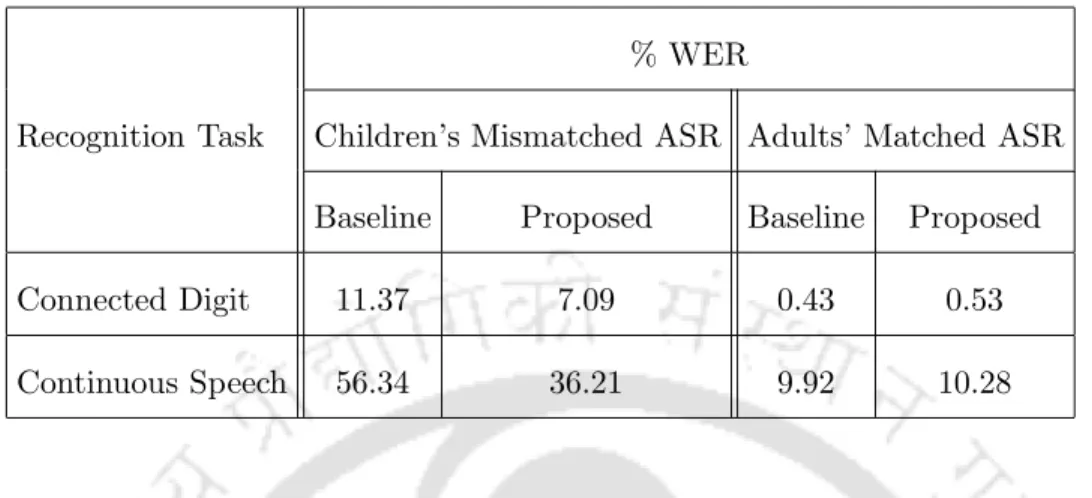
Combining Proposed Algorithm with VTLN and CMLLR
The recognition performance for the child test set PFts on the adult and child speech-trained models using the standard MFCC features and the features derived using the proposed adaptive MFCC feature truncation algorithm, both with and without CMLLR, are also given in Table 7.12. It is noted that large relative gains of 40% and 26% are achieved in children's ASR performance when doing CMLLR using MFCC features derived using the proposed algorithm, compared to the performance obtained by doing CMLLR using the standard MFCC features on the speech-trained and speech-oriented features of adults. the speech-trained child models, respectively.

Summary
Similar study can therefore be investigated to address the acoustic mismatch for children's ASR which is expected to further improve the performance of the children's speech recognition on adult's speech trained models. Pelton, “Identifying pronunciation errors in children's speech: Exploring the role of the speech recognizer,” in Proc.