It has been a privilege of my career to be involved in many information retrieval evaluation campaigns. NTCIR NACSIS/NII Collection for Information Retrieval Systems/NII Test States and Community Research for Access to Information.
Graded Relevance
- Introduction
- Graded Relevance Assessments, Binary Relevance Measures
- Early IR and CLIR Tasks (NTCIR-1 Through -5)
- Patent (NTCIR-3 Through-6)
- Math/MathIR (NTCIR-10 Through -12)
- Graded Relevance Assessments, Graded Relevance Measures
- Web (NTCIR-3 Through-5)
- CLIR (NTCIR-6)
- ACLIA IR4QA (NTCIR-7 and -8)
- GeoTime (NTCIR-8 and -9)
- CQA (NTCIR-8)
- INTENT/IMine (NTCIR-9 Through 12)
- RecipeSearch (NTCIR-11)
- Temporalia (NTCIR-11 and -12)
- STC (NTCIR-12 Through -14)
- WWW (NTCIR-13 and -14) and CENTRE (NTCIR-14)
- AKG (NTCIR-13)
- OpenLiveQ (NTCIR-13 and -14)
- Summary
The estimated levels of importance were defined as follows: A (a citation that was actually used alone to reject a given patent application) and B (a citation that was actually used together with another to rejected a certain patent application). The Document Retrieval (DR) subtask of the INTENT task had purposefully rated importance ratings on a 5-point scale.
Eguchi K, Oyama K, Ishida E, Kando N, Kuriyama K (2003) Overview of the web fetch task during the third NTCIR workshop. Zeng Z, Kato S, Sakai T (2019) Overview of the NTCIR-14 Short Text Conversational Task: Dialog Quality and Nugget Detection Subtasks.
Information Retrieval Using Comparable Corpora of Chinese, Japanese,
- Introduction
- Outline of Cross-Language Information Retrieval (CLIR)
- CLIR Types and Techniques
- Word Sense Disambiguation for CLIR
- Language Resources for CLIR
- Test Collections for CLIR from NTCIR-1 to NTCIR-6
- Japanese-English Comparable Corpora in NTCIR-1 and NTCIR-2
- Chinese-Japanese-Korean (CJK) Corpora from NTCIR-3 to NTCIR-6
- CJKE Test Collection Construction
- IR System Evaluation
- CLIR Techniques in NTCIR
- Monolingual Information Retrieval Techniques
- Bilingual Information Retrieval (BLIR) Techniques
- Multilingual Information Retrieval (MLIR) Techniques
- Concluding Remarks
Monolingual IR was specifically referred to as monolingual IR (SLIR) in the NTCIR CLIR tasks. The resulting SLIR performance improvement can be viewed as an achievement in the NTCIR CLIR tasks.

Text Summarization Challenge
An Evaluation Program for Text Summarization
- What is Text Summarization?
- Various Types of Summaries
- Evaluation Metrics for Text Summarization
- Text Summarization Evaluation Campaigns Before TSC
- TSC: Our Challenge
- TSC1
- TSC2
- TSC3
- Text Summarization Evaluation Campaigns After TSC
- Future Perspectives
Text summarization is a task of producing a shorter text from the source while preserving the information content of the source. Recall: determines what proportion of the sentences selected by humans are selected by the system.
Challenges in Patent Information Retrieval
Introduction
Since patent applications are highly technical and their length tends to be long, the task of searching patent applications poses many information retrieval issues. The NTCIR tasks were created based on current patent-related work involving a large number of patent applications.
Overview of NTCIR Tasks .1 Technology Survey
- Invalidity Search
- Classification
- Mining
For the construction of the map, we focused on the basic (underlying) technologies used in a particular field and their effects. Knowledge of the history and effects of the basic technologies used in a particular field.
Outline of the NTCIR Tasks
- Technology Survey Task: NTCIR-3
- Search Topics
- Document Collections
- Submissions
- Relevance Judgments
- Evaluation
- Participants
- Invalidity Search Task: NTCIR-4, NTCIR-5, and NTCIR-6
- Search Topics
- Document Collections
- Submissions
- Relevance Judgments
- Evaluation
- Participants
- Patent Classification Task: NTCIR-5, NTCIR-6
- Data Collections
- Evaluation
- Participants
- Patent Mining Task: NTCIR-7, NTCIR-8
- Research Paper Classification Subtask
- Technical Trend Map Creation Subtask Task
We used 378 relevant applications obtained from 34 search subjects of NTCIR-4 main subjects plus another 6 that had been used in the trial in NTCIR-4. In the NTCIR-5 passage retrieval task, we reused the search topics in NTCIR-4 and all relevant passages were collected in NTCIR-4 by JIPA members. Each participant in NTCIR-5 submitted a ranked list of themes (maximum 100) for each test document in the theme classification task and a ranked list of F terms (maximum 200) for each test document in the F term classification task.

Contributions
- Preliminary Workshop
- Technology Survey
- Collaboration with Patent Experts
- Invalidity Search
- Patent Classification
- Mining
- Workshops and Publications
- CLEF-IP and TREC-CHEM
In: Proceedings of the 5th NTCIR workshop on research in information access technologies information retrieval, question answering and summarization. In: Proceedings of the 6th NTCIR workshop meeting on evaluation of information access technologies: information retrieval, question answering and cross-language information access. In: Proceedings of the 5th NTCIR workshop meeting on evaluation of information access technologies: information retrieval, question answering and cross-language information access.
Multi-modal Summarization
- Background
- Applications Envisioned
- Multi-modal Summarization on Trend Information
- Objective
- Data Set as a Unifying Force
- Outcome
- Implication
The objective of the MuST workshop was to create an agora or arena where scholars from the several fields mentioned above can interact. The author also thanks all the workshop participants for their valuable research efforts on multi-modal summarization. In: Proceedings of the 5th meeting of the NTCIR Workshop on Evaluation of Information Access Technologies, p. 556–563.
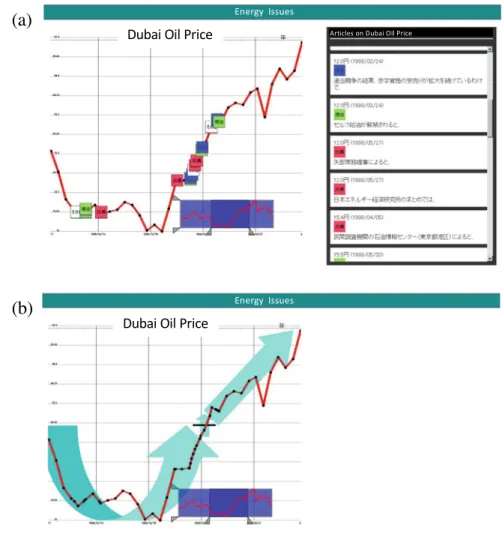
Opinion Analysis Corpora Across Languages
- Introduction
- NTCIR MOAT
- Overview
- Research Questions at NTCIR MOAT
- Subtasks
- Opinion Corpus Annotation Requirements
- Cross-Lingual Topic Analysis
- Opinion Analysis Research Since MOAT
- Research Using the NTCIR MOAT Test Collection
- Opinion Corpus in News
- Current Opinion Analysis Research: The Social Media Corpus and Deep NLP
- Conclusion
I: Proceedings of the 49th annual meeting of the association for computational linguistics (ACL 2011), Portland, Oregon, s. 151-160. I: Proceedings of the 49th annual meeting of the association for computational linguistics (ACL 2011), Portland, Oregon, pp. 320-330. I: Proceedings of the 43rd annual meeting of the association for computational linguistics (ACL 2005), Ann Arbor, Michigan, pp. 115-124.

Patent Translation
Introduction
Workshop on Statistical Machine Translation (WMT) (2006 to present (2019) see footnote 1): Machine translation between European languages is the goal. In 2007, RBMT systems entered the market for machine translation of patents between Japanese and English. Before 2007, however, there were few studies on corpus-based machine translation for patents.
Innovations at NTCIR
- Patent Translation Task at NTCIR-7 (2007–2008)
- Patent Translation Task at NTCIR-8 (2009–2010)
- Patent Translation Task at NTCIR-9 (2010–2011)
- Patent Translation Task at NTCIR-10 (2012–2013)
The organizers3 added a Chinese-English patent translation assignment in addition to the Japanese-English and English-Japanese patent translation assignments. Japanese-English, English-Japanese, and Chinese-English patent translation tasks continued at NTCIR-10. For Japanese-English translations, RBMT was still better than SMT; however, the translation quality of the best SMT system was improved over NTCIR-9 (Sudoh et al.2013).
Developments After NTCIR-10
7 Patent Translation 107 Nakazawa T, Mino H, Goto I, Neubig G, Kurohashi S, Sumita E (2015) Overview of the 2nd Workshop on Asian Translation. Nakazawa T, Ding C, Mino H, Goto I, Neubig G, Kurohashi S (2016) Overview of the 3rd Workshop on Asian Translation. In: Proceedings of the 3rd workshop on Asian translation (WAT2016), COLING 2016 Organizing Committee, Osaka, Japan, pp. 1-46.
Component-Based Evaluation for Question Answering
- Introduction
- History of Component-Based Evaluation in QA
- Contributions of NTCIR
- Component-Based Evaluation in NTCIR
- Shared Data Schema and Tracks
- Shared Evaluation Metrics and Process
- Recent Developments in Component Evaluation
- Open Advancement of Question Answering
- Configuration Space Exploration (CSE)
- Component Evaluation for Biomedical QA
- Remaining Challenges and Future Directions
- Conclusion
Therefore, we developed a component-based evaluation approach for error analysis and improvement of the JAVELIN CLQA system (Lin et al. 2005; Shima et al. 2006). In: Kando N (ed) Proceedings of the 6th NTCIR Workshop Meeting on Evaluation of Information Access Technologies: Information Retrieval, Question Answering and Cross-Linguistic Information Access, NTCIR-6. In: Kando N (ed) Proceedings of the 7th NTCIR Workshop Meeting on Evaluation of Information Access Technologies: Information Retrieval, Question Answering and Cross-Linguistic Information Access, NTCIR-7.

Temporal Information Access
- Introduction
- Temporal Information Retrieval
- NTCIR-8 GeoTime Task
- NTCIR-9 GeoTime Task Round 2
- Issues Discussed Related to Temporal IR
- Temporal Query Analysis and Search Result Diversification
- NTCIR-11 Temporal Information Access Task
- NTCIR-12 Temporal Information Access Task Round 2
- Implications from Temporalia
- Related Work and Broad Impacts
- Conclusion
An example of the query is "When and where was the location of the host city for the 2010 Winter Olympics announced?". In the query discussed above, "2010 Winter Olympics" is the name of the event and can be treated as an implicit temporal expression. In this method, the proximity of the geographical and temporal information is taken into account for ranking documents in addition to the standard ranking of information retrieval such as BM25.

SogouQ: The First Large-Scale Test Collection with Click Streams Used in a
- Introduction
- SogouQ and Related Data Collections
- SogouQ and NTCIR Tasks
- Impact of SogouQ
- Conclusion
In the Sub-Topic Mining subtask, a sub-topic can be a specific interpretation of an ambiguous query (eg, "microsoft windows" or "home windows" in response to . "windows") or an aspect of a facet query (eg, "windows 7" update" in response to "windows 7"). The NTCIR-11 IMine task continued the sub-theme mining sub-task and the document ranking sub-task and started a sub -new task called TaskMine, which aims to explore methods of automatically finding subtasks of a given task (eg, for a given task "lose weight", Possible results might be "do physical exercise ", "get calories", "take diet pills", etc.). For example, given the ambiguous query "windows", the first-level subtopic might be "microsoft windows", "software on windows platform", or "house windows".
Evaluation of Information Access with Smartphones
- Introduction
- NTCIR Tasks for Information Access with Smartphones
- NTCIR 1CLICK
- NTCIR MobileClick
- Evaluation Methodology in NTCIR 1CLICK and MobileClick
- Textual Output Evaluation
- From Nuggets to iUnits
- S-Measure
- M-Measure
- Outcomes of NTCIR 1CLICK and MobileClick
- Results
- Impacts
- Summary
In the NTCIR 1CLICK and MobileClick tasks, more important information is expected to be present at the beginning of the summary so that users can reach such information efficiently. This section provides a brief overview of the job design of the NTCIR 1CLICK and MobileClick jobs. The history of accessing information with smartphones in NTCIR began with a subtask of the NTCIR-9 INTENT task, namely NTCIR-9 1CLICK-1 (formally one-click access task) (Sakai et al.2011b).
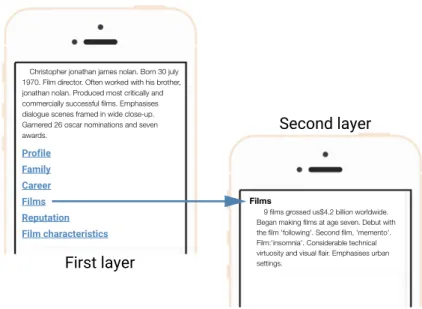
Mathematical Information Retrieval
Introduction
Schwarz Hölder", but that keyword was the crucial information the engineer missed in the first place. The formula structure is mapped by unification (finding a substitution for the boxed query variables to make the query and main formula of Hölder's inequality structurally identical or similar (see Section 12.3.2). We used the context information about the parameters of Hölder's inequality, for example that the identifiers f,g,p and q are universal (i.e. can be replaced by);.
NTCIR Math: Overview
- NTCIR-10 Math Pilot Task
- NTCIR-11 Math-2 Task
- NTCIR-12 MathIR Task
In the NTCIR-11 Math-2 task (Aizawa et al. 2014), both the corpus and arXiv topics were reconstructed based on feedback from participants in the pilot task. Eight teams participated in the NTCIR-11 Math-2 task (two new teams joined), and most contributed to both subtasks. For the NTCIR-12 MathIR task (Zanibbi et al.2016), we reused the arXiv corpus prepared for the NTCIR-11 Math-2 task, but with new topics.
NTCIR Math Datasets
- Corpora
- ArXiv Corpus
- Wikipedia Corpus
- Topics
- Topic Format
- ArXiv Topics
- Wikipedia Topics
- Relevance Judgment
The arXiv corpus contains paragraphs from technical articles in arXiv2, while the Wikipedia corpus contains full articles from Wikipedia. These statistics show that the mathematical trees in the arXiv corpus roughly follow the power distribution in size. The corpus contains more than 590,000 formulas in the same format as the arXiv corpus, i.e. coded using LATEX, Presentation MathML and Content MathML.

Task Results and Discussion .1 Evaluation Metrics
- MIR Systems
- How to Index Math Formulae?
- How to Deal with Query Variables?
- Other Technical Decisions
One of the salient features of MIR is that a query formula can contain "variables," i.e., symbols that can serve as named characters. Since the unification operation is expensive, most participating systems used a reorder step, where one or more initial orders are merged and/or reordered. To find strong partial matches, all automated systems used unification, whether for variables (eg, "x2+y2=z2" unifies "a2+b2=c2"), constants, or entire subexpressions ( (eg, through structural unification or indirectly through generalized wildcard terms for operator arguments).
Further Trials
- ArXiv Free-Form Query Search at NTCIR-10
- Wikipedia Formula Search at NTCIR-11
- Math Understanding Subtask at NTCIR-10
The goal of the Math Understanding subtask was to extract natural language definitions of mathematical formulas in a document for their semantic interpretation. For example, in the text "log(x) is a function that calculates the natural logarithm of the value", the complete description of "log(x)" is "a function that calculates the natural logarithm of the value". To calculate F1 scores, the positions of the annotated descriptions from two annotators are carefully matched.
Further Impact of NTCIR Math Tasks
- Math Information Retrieval
- Semantics Extraction in Mathematical Documents
- Corpora for Math Linguistics
For example, Kristianto et al. 2017) combined description extraction with formula dependency extraction and achieved consistent improvement in math acquisition subtasks in subsequent NTCIR math tasks. The problem is that many mathematical corpora (eg the arXiv corpus or the 3 Million abstracts of zbMATH12) are not available under a license that allows re-publication. The main example dataset is the XHTML5+MathML version of the arXiv corpus as of August 2019.13.
Conclusion
In: Proceedings of the 38th ACM SIGIR International Conference on Research and Development in Information Retrieval. In: Proceedings of the 53rd Annual Meeting of the Association for Computational Linguistics and the 7th International Joint Conference on Natural Language Processing (Volume 2: Short Papers), Association for Computational Linguistics, Beijing, China, p. 334–340.https:// doi.org/10.3115/v1/P15-2055. In: Proceedings of the 2018 Conference of the North American Division of the Association for Computational Linguistics: Human Language Technologies, Vol. 1 (Long Papers), Association for Computational Linguistics, New Orleans, Louisiana, p. 303–312.https://doi .
Introduction
Related Activities
In terms of actual functional retrieval systems for life log data, a number of early retrieval engines had been developed prior to NTCIR-12, such as the MyLifeBits system (Gemmell et al. 2002) or the Sensecam Browser (Lee et al. 2008). The NTCIR-12 Lifelog pilot task (Gurrin et al.2016) introduced the first shared test collection for lifelog data and attracted the first cohort of participants to what was at the time a very novel and challenging task. Once again, we refer the reader to (Gurrin et al. 2014b) for an overview of early efforts in life log search and retrieval.
Lifelog Datasets Released at NTCIR
Human activity data: The daily activities of the lifeloggers were captured in terms of the semantic locations visited, physical activities (e.g. walking, running, standing) from the Moves application, 4 together with (for NTCIR-14) a time-stamped diet log of all food and drink consumed. Improvements to the data: The wearable camera images were annotated with the outputs of various visual concept detectors that described the content of the life log images in text form. Before release, each data set was subject to a detailed multi-phase redaction process to anonymize the data set in terms of the life registrant's identity as well as the identity of bystanders in the data.
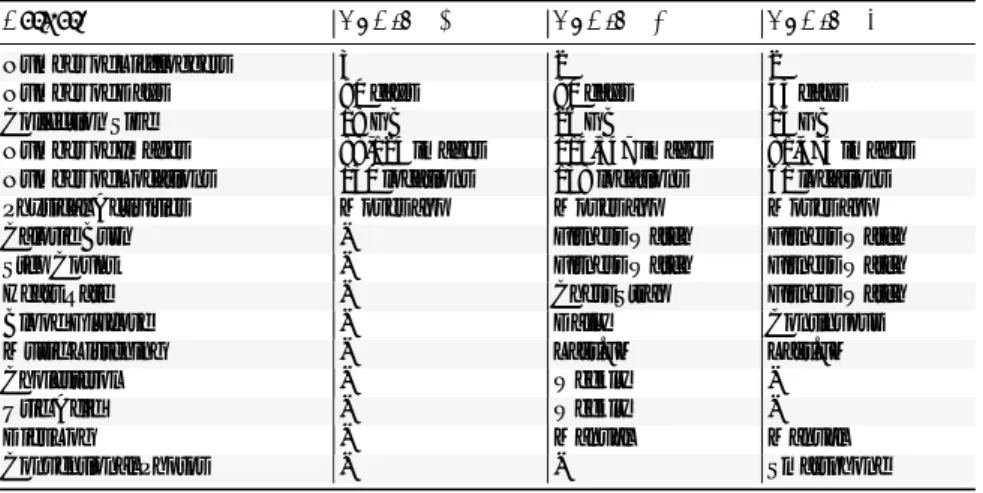
Lifelog Subtasks at NTCIR
- Lifelog Semantic Access Subtask