Multi-step-ahead forecast errors will be serially correlated, even if the forecasts are optimal, due to the forecast-period overlap associated with multi-step-ahead forecasts. Tests based on the first autocorrelation (eg, the Durbin-Watson test), as well as more general tests, such as the Box-Pierce and Ljung-Box tests, are also useful. 1Since in many applications the loss function will be a direct function of the prediction error, L(yt, yt+h,t) = L(et+h,t), we write L(et+h,t) from this point to save on notation, while recognizing that certain loss functions (such as direction-of-change) do not collapse to the L(et+h,t) form.
Error variance measures the spread of the predicted errors, which is another component of accuracy. For example, many economic variables may in fact be almost random walks, in which case forecasters will have great difficulty beating the random walk through no fault of their own (i.e., the predictive R2 relative to a random walk “no change” prediction given by The U of Theil may be located near 0). The loss function L(·) need not be quadratic or even symmetric; we only require that L(0) = 0 and that L(·) is strictly monotonic on each side of the origin.
Clearly, parametric measures of predictability will generally depend on the specification of the parametric model. The DM is simply an asymptotic z-test of the hypothesis that the mean of a constructed but observed series (the loss difference) is zero. Therefore, the standard error in the denominator of the DM statistic (10.3) should be robustly calculated.
The key is to recognize that the DM statistic can be trivially calculated by regressing the loss difference on an intercept, using heteroscedasticity and autocorrelation robust (HAC) standard errors.
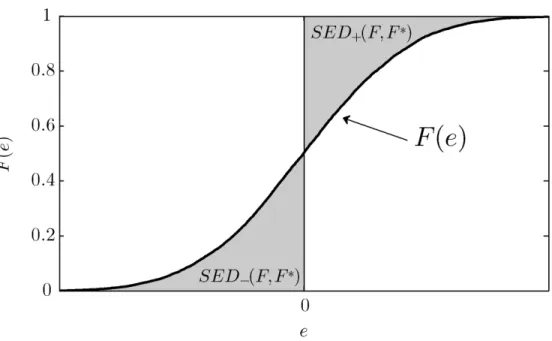
OverSea Shipping
In Figures 3 and 4 we plot the errors from the quantitative and judgmental forecasts, which are more revealing. In particular, the quantitative error appears roughly centered on zero, whereas the judgment error appears to be slightly higher than zero on average. This means that the judgmental forecast appears biased in a pessimistic way - on average, the actual realized volume is slightly higher than the expected volume.
In Figures 5 and 6, we show histograms and related statistics for the quantitative and judgmental forecast errors. The histograms confirm our earlier suspicions based on error plots; the histogram for the quantitative error is centered on a mean of -.03, whereas the histogram for the judgmental error is centered on 1.02. However, the error standard deviations reveal that the estimated forecast errors vary somewhat less around their mean than the quantitative errors.
In Tables 1 and 2 and Figures 7 and 8 we show the correlograms of the quantitative and judgmental forecast errors. We show the results for the quantitative forecast errors in Table 3, and those for the judgmental forecast errors in Table 4. The t-statistic indicates no bias in the quantitative forecasts, but considerable and highly statistically significant bias in the judgmental forecasts.
We expected the judgmental forecast to fail because it is biased, but so far no errors have been found in the quantitative forecast. We show the histogram and descriptive statistics for the squared quantitative and judgment errors in Figures 9 and 10. The histogram for the squared judgment error is shifted to the right relative to the quantitative error due to bias.
The RM SE of the quantitative prediction is 1.26, while the judgment value is 1.48. In Figure 11 we show the (squared) differential loss; it is quite small but looks a little negative. Figure 12 shows the histogram of the loss differential; the mean is -.58, which is small relative to the standard deviation of the difference in losses, but remember that we have not yet corrected for serial correlation.
To test for the significance of the loss differential, we regress it on a constant and allow for M A(1) disturbances; we show the results in Table 8. The mean loss difference is highly statistically significant with a p-value less than 0.01; we conclude that the quantitative forecast is more accurate than the judgmental forecast under quadratic loss.
Exercises, Problems and Complements
For the following, use the shipping volume time series, quantitative forecasts, and judgment forecasts used in this chapter. Using the first 250 weeks of shipment volume data, specify and estimate a univariate autoregressive shipment volume model (with trend and seasonality if necessary) and provide evidence to support the appropriateness of your chosen specification. What are the advantages and disadvantages of home experts vs. c) Kaggle unusually allows people to peek at the test sample by resubmitting predictions once a day.
Suppose someone assigns a very high probability to an event that does not occur, or a very low probability to an event that does occur. Even events that are correctly predicted to happen with a high probability simply cannot happen, and vice versa. So, for example, a currency may sell forward at a large discount, indicating that the market has assigned a high probability of a large depreciation.
In the event that depreciation may fail, this does not necessarily mean that the market was in any sense "wrong" in assigning a high probability of depreciation. Some optimality tests can be obtained even when the prediction target is unobservable (Patton and Timmermann 2010, based on Nordhaus 1987). Thus, the autoregressive model can be viewed as a sieve, so our approach is actually nonparametric.
For each fixed sample size, we estimate predictability through the lens of a particular autoregressive model. Therefore, it may be of interest to develop an approach with a more fully non-parametric flavor by exploiting the well-known Kolmogorov spectral formula for the univariate innovation variance. Most of the basic lessons for estimating time series forecasts presented in this chapter are also relevant to cross-sectional forecast estimation.
DM type tests can be done for point predictions, and DGT type test for density predictions. As we have shown, Mincer-Zarnowitz corrections can be used to “correct” sub-optimal point predictions. They can also be used to produce density forecasts, drawing from an estimate of the density of the MZ regression perturbations, as we did in another context in section 4.1.
Notes