To the participants and everyone at one SA SME who took part in this study, I thank you for your time, your support and for sharing your knowledge and experience with me. To my brother, Collen Mawela, thank you for always being there for me when I needed you most.
Introduction
It further analyzes the literature to determine the challenges of poor data quality and benefits of high data quality. The research purpose and objectives are presented in sections 1.4.1 and 1.4.2, followed by the purpose of the research (section 1.4.3) and research questions in section 1.4.4.
Background to study
Data quality requires an understanding of which dimensions of data quality are critical for the user (Wang et al., 1992:4). The concept of data quality is divided into four dimensions; accuracy, timeliness, completeness and consistency (Haug et al Singh & Vashishtha, 2015:182).
Problem statement
SMEs need better storage systems, improved data management capabilities, efficient data acquisition, and agile analysis and reporting that can assist management in their decision-making processes (Guarda et al. However, SMEs struggle to use their business data to gain information insight that will enable them making informed decisions, measuring performance, innovating, reducing costs, analyzing customer trends and gaining a competitive advantage over their rivals (Curraj, 2018:17; Guarda et al. Papachristodoulou et al. Ponis & Christou, 2013:4; Razbonyalı & Güvenoğlu.
Research objectives
- Theoretical objectives
- Practical objectives
- The research purpose
- Research questions
How can SMEs leverage technology to better manage data and improve the quality of their data for data analysis. SQ3: What are the challenges of poor data quality and the benefits of high data quality in SMEs.
Research methodology
- Design science research
- Design science research applied to this study
- Participants
- Data collection and analysis
- Ethical considerations
- Rigour of the study
- Assumptions and limitations of the study
Awareness of the problem at this stage focused on the data management and quality needs of one SA MSP. Unit of analysis refers to “the basic unit of text to be classified during content analysis” (Zhang & Wildemuth, 2009:3).
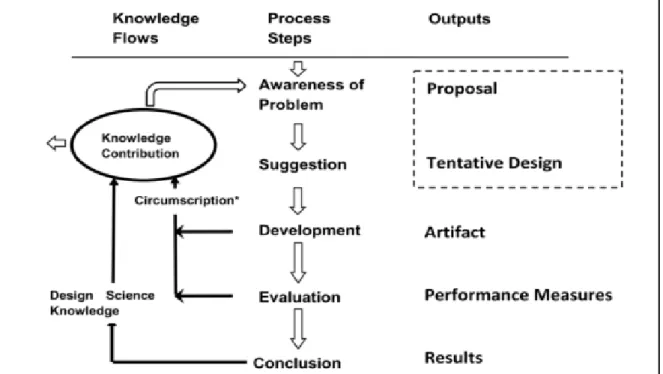
Significance of the study
The main limitation of this study is that it was based on one SA SME, and the guidelines to improve data quality and data management were developed based on the needs of this SME. Another limitation would therefore be the limited number of participants interviewed and the small number of responses from questionnaires.
Dissertation layout
This chapter describes the key concepts of the study, providing in-depth explanations of data quality, data management, business intelligence, data warehouse, data analytics, and ETL. In addition, the case environment is also explained to gain insight into the SME involved in the study.
Conclusion
LITERATURE REVIEW
Introduction
Data
Information
Knowledge
Wisdom
Data quality
- Data quality dimensions
- Data quality methodologies
- Big data
- Poor data quality
Data management
Small and Medium-sized Enterprises
Data analytics
- Data warehousing and business intelligence
- ETL
Related Study
Conclusion
RESEARCH METHODOLOGY
Introduction
Research paradigms
- Positivism
- Critical social research
- Interpretivism
- Design science research
- Positioning the study within DSR
Research approaches
- Qualitative research
- Quantitative research
- Mixed method research
Research strategies
- Ethnography
- Grounded theory
- Action research
- Case study
Data collection techniques
- Interviews
- Questionnaires
- Focus groups
- Observations
Data analysis
Design science research
- The design science research framework
- Guidelines for design science research in information systems
Three design science research models
- Design science research cycles (Peffers et al., 2007:48)
- Design science research cycles (Hevner & Chatterjee, 2010:16)
- Design Science Research Process Model (Vaishnavi et al., 2004/2019:14)
- Preferred research model for the study
Evaluation of design science research artefacts
Ethics in design research
Study plan
Rigour of the study
Conclusion
DESIGN AND DEVELOPMENT
Introduction
- Awareness phase
- Suggestion phase
Designing the artefact
- Research sub-cycle 1: Literature review
- Research sub-cycle 2: Literature review
- Case study environment
- Research sub-cycle 3: Exploratory study
Development of artefact
Conclusion
DEMONSTRATION AND EVALUATION
Introduction
In this chapter, the researcher will discuss the artifact implementation process, the results and findings of the study. In the process, the researcher will address the practical objectives of the research study and the plan the researcher followed in answering the research questions in order to determine a solution to the research problem. A case study research strategy was followed to gain an in-depth understanding of the problem and to construct a solution to meet one SA SME's data quality and management needs.
Mixed method data collection techniques such as interviews, observation and a questionnaire were used to collect data from the participants. Participants' knowledge, personal experiences and involvement in the study contributed to the success of the research study. Information obtained from the literature, as well as research study findings and results were used to compile the guidelines and technologies needed to improve data quality and management in one SA SME.
The researcher will discuss the results of the research study in section 5.5 where the themes and codes used to analyze data using ATLAS.ti are discussed.
Participants
In this study, the researcher selected a sample that is unbiased and can provide in-depth and accurate information needed to understand the phenomena and answer the research questions. An appropriate sampling technique to consider when selecting a sample for this study is purposive sampling because the researcher was already aware of where the participants would be drawn from and believed that these participants would provide a wealth of knowledge about the phenomena or situation. A senior manager recommended the IT administrator and data capture staff to participate in this study because he believed they would provide the researcher with key information needed in this study.
The IT administrator and the data capturer agreed to participate in the research study to share their knowledge with the researcher. The data capturers, IT administrator and IT manager have extensive knowledge about the phenomena, the situation, the current data management architecture, the organization's technical architecture and data quality issues (section 4.2.3) . These participants can provide exceptional insight into the current data management infrastructure, the challenges and limitations presented, and the needs of SMEs.
The IT manager was selected to provide in-depth knowledge of the IT infrastructure of SMEs and to share more insight on the phenomena and issues from a technical perspective in a broader context. This participant's experience and knowledge gave the researcher a better understanding of the problem in a broader context, the constraints and challenges from a business perspective, and in-depth knowledge of the SME's business processes, functionalities and decision-making processes.
Evaluation – main research cycle
The senior manager was selected to provide insight and extensive knowledge of the phenomena and role of data management architecture in business management, process modeling and decision making. The researcher designed these test cases to evaluate the artifact's functionality, accessibility, simplicity and elegance, ease of use, visibility, readability, efficiency, completeness, data accuracy, security, and understandability. Receive Some text boxes, dropdown lists, and buttons need to be scaled to fit the text properly.
Dropdown list, text box and labels were enlarged to ensure the text fits correctly, is visible and readable.
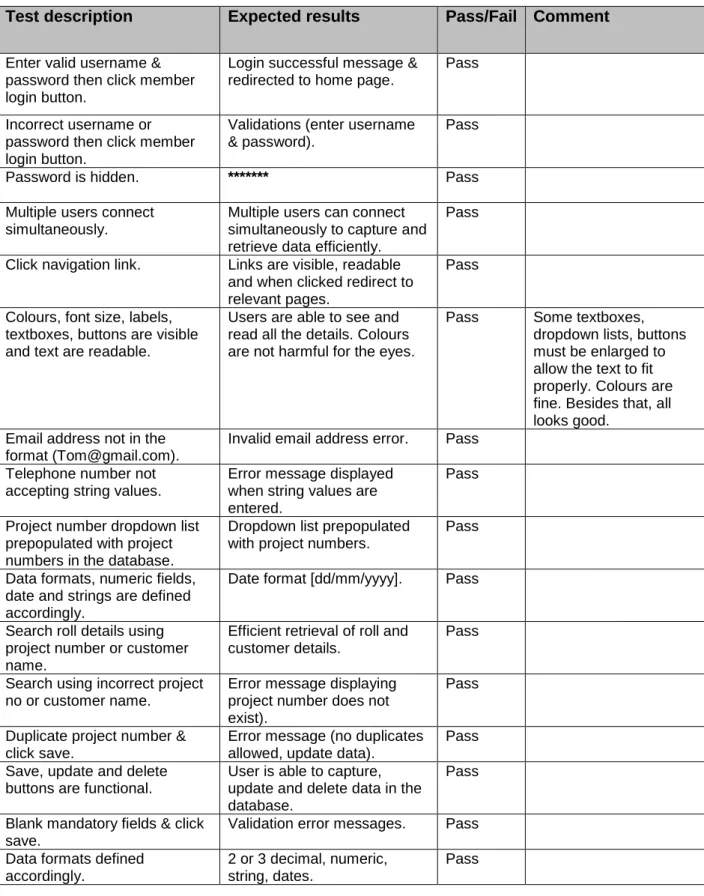
Data collection
- Observation
- Questionnaires
- Semi-structured interviews
When formulating the questionnaire questions, the researcher took into account the guidelines described in section 3.5.2 to ensure that the participants did not feel frustrated, embarrassed or uncomfortable in answering the questions. The researcher chose semi-structured interviews as the data collection technique because it provided a level of flexibility in how the researcher asked the questions, and also allowed the participants to tell their story and discuss issues with the researcher (section 3.5.1) . Based on the data quality and management literature, the researcher formulated interview questions to ask the two participants about the newly implemented artifact data capture and management functionality.
The researcher ensured that the interview questions were formulated as described in Appendix 2 to obtain all the necessary required information. The researcher sent an email to the IT administrator and the data capturer requesting an interview session with them. Additionally, the researcher asked to record the interview session and explained to the participants that all their personal information will be kept confidential and the data collected during the interview process will be transcribed and used only for research analysis and conclusions and will stored in a secure location for a period of five years as discussed in section 3.10.
The researcher ensured that the interview was conducted professionally, in an ethical manner, ensuring that the interests of the participants were well protected from any form of harm. Semi-structured interviews enabled the researcher to gain insight into the experiences, thoughts, behavior and understanding of one of the SA SME participants about the artefact.
Results of the research
The participants' involvement in the evaluation stages as described in section 5.2 helped the researcher to identify problems and complications in the artefact. The following question was used to obtain more feedback on the operability and ease of use of the artifact. The following question was used to obtain feedback on user expression and thoughts on the functionality of the artifact.
In this section, the researcher interviewed the participants about their overall impression regarding the functionality of the artifact. The participants indicated that they found the artifact very useful and commented on the value added to the organization. As detailed in Figure 5-4, the results based on user satisfaction about the functionalities of the artifact indicated that the participants were satisfied with the artifact functionalities.
The results of the SSPS indicated that the participants were satisfied with the artifact and that they found the artifact pleasant to use. Compared to the old data management solution, the artifact allowed users to connect and capture data simultaneously.
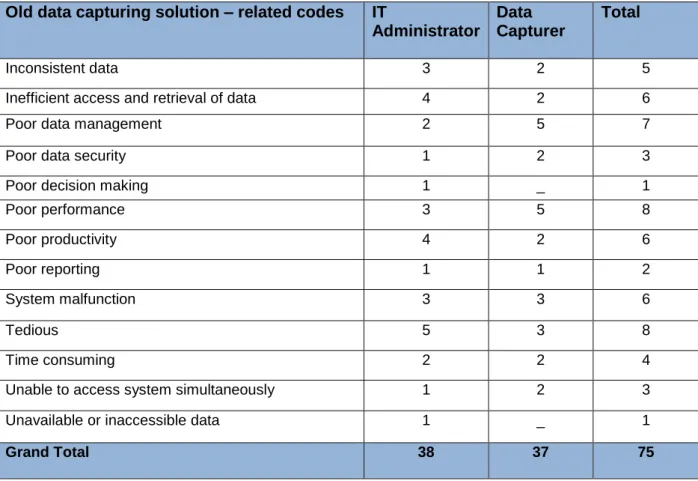
Guidelines and technologies for improvement of data quality in one South African
It is the responsibility of senior management to establish enterprise-wide data quality awareness programs and data quality training. An individual is required capable of managing data security, performing data quality checks, monitoring system functionality and providing data support when required. Data profiling involves examining data quality issues within existing data and determining corrective actions to be taken.
Dimensions such as accuracy, validity, timeliness, consistency, uniqueness and completeness can be used to assess and measure data quality. Using data quality dimensions can increase the level of data quality and thus bring benefits to the organization. Data quality tools play an important role in helping SMEs capture, store, manage and analyze data.
Technologies that one SA SME can use to improve data quality and analytics are then discussed. These tools can enable one SA SME to improve data quality and develop a data-driven business model that will enable the company to gain a competitive advantage, increase revenue and improve decision-making processes.
Conclusion
RESEARCH CONCLUSIONS AND RECOMMENDATIONS
- Introduction
- Research objectives addressed
- Theoretical objective
- Practical objectives
- Theoretical and practical contributions
- Conclusion phase – Main research cycle
- Rigour of the research
- Limitations of the study
- Recommendations for future research
- Reflection and conclusion
Poor data quality in a SA SME led to poor decision making, mistrust of organizational data, poor performance and put the SME at a competitive disadvantage. Theoretical objective 3: To identify the challenges of poor data quality and the benefits of high data quality in SMEs. In one SME, poor data quality caused mistrust in organizational data, reporting and decision-making processes.
Practical objective 2: to propose guidelines and technologies for improving data quality in a SA SME. Top management in a SA SME should educate or train employees about the importance of data quality in the organization, especially its impact on decision-making processes. Senior managers in a SA SME must develop a data-driven culture and mindset necessary to manage and maintain data quality.
ETL tools can be used to integrate, cleanse and transform data to improve data quality. In addition, organizations can follow the five phases of the Six Sigma DMAIC approach to improve data quality.
EXPLORATORY STUDY – QUESTIONS
POST IMPLEMENTATION INTERVIEW QUESTIONS
QUESTIONNAIRE (POST IMPLEMENTATION)
DATA TRANSFORMATION SSIS PACKAGE
DW STAR SCHEMA
SQL SERVER REPORTING AND ANALYTICS
OLD DATA MANAGEMENT CODE NETWORK
NEW ARTEFACT CODE NETWORK
NEW REPORTING SOLUTION CODE NETWORK
SUPPORTING CODE
CERTIFICATE OF EDITING